Decoding Risk: How the Boosted Difference of Convex Functions Algorithm is Revolutionizing Portfolio Optimization
"Unlock superior returns and navigate financial uncertainty with the BDCA – the cutting-edge tool reshaping Value-at-Risk constrained portfolio management."
For over seven decades, the challenge of refining portfolio selection, initially framed by Markowitz's Mean-Variance (MV) model in 1952, has captivated financial minds. The field has evolved into two mainstreams: enhancing risk assessment through diverse measures and integrating more complex financial realities and constraints. Current strategies often struggle to balance real-world applicability and the need to accurately reflect the intricate nature of today's financial landscape.
Contemporary financial institutions, governed by strict regulations, rely on Value-at-Risk (VaR) to manage exposures across credit, market, and operational domains. However, integrating VaR into portfolio optimization is complicated by its mathematical properties; traditional methods often fall short in delivering optimal solutions within reasonable time frames.
This article explores the potential of the Boosted Difference of Convex Functions Algorithm (BDCA) in addressing these challenges. By achieving faster convergence and improved solutions, BDCA promises to refine how financial professionals construct and manage portfolios under VaR constraints. We’ll explore the algorithm's mechanics, performance, and practical implications for the modern investor.
What is Value-at-Risk (VaR) and Why is it so Difficult?
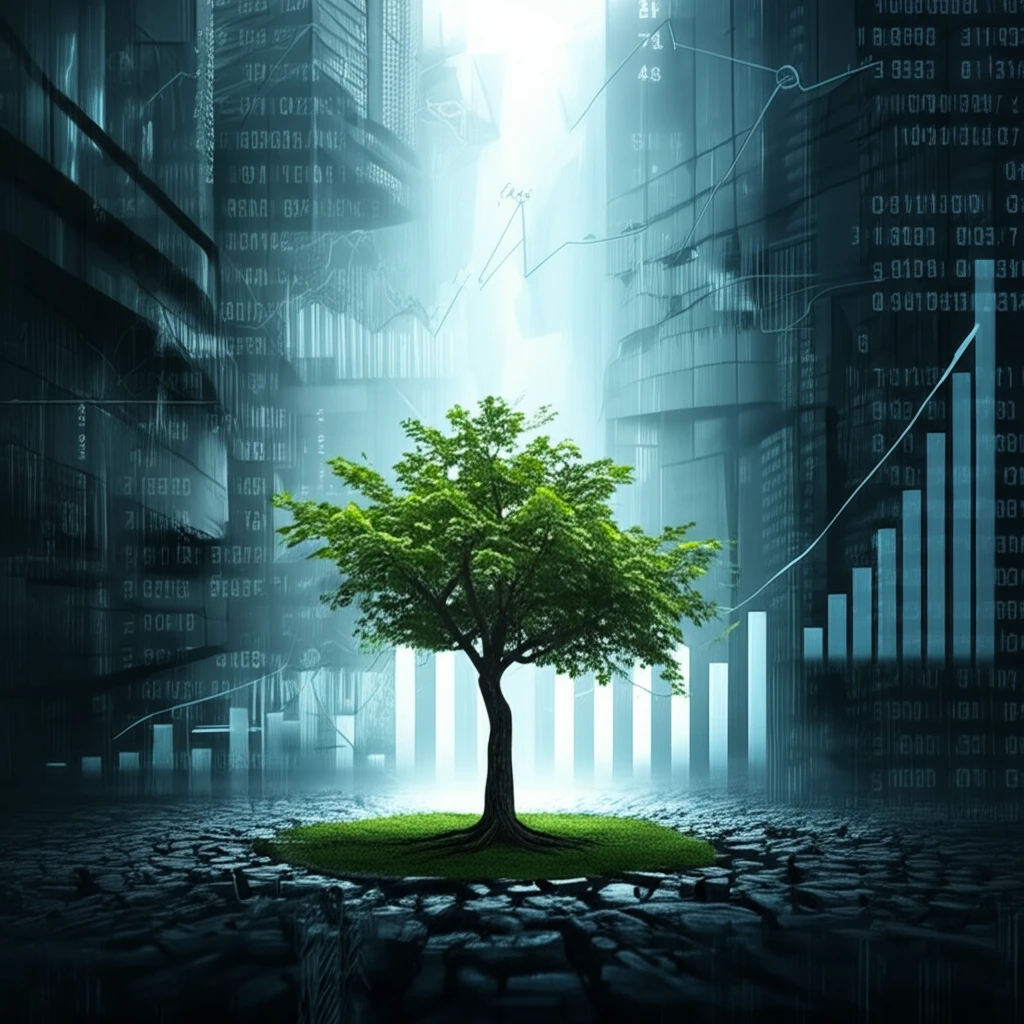
Value-at-Risk (VaR) represents the maximum expected loss over a specified time horizon at a given confidence level. Financial institutions use it to determine the amount of capital they need to reserve to cover potential losses. While crucial for regulatory compliance and internal risk management, VaR's mathematical properties pose significant challenges for portfolio optimization.
- Non-Convexity: VaR is not a mathematically "well-behaved" function, making it difficult to incorporate into standard optimization frameworks.
- Computational Complexity: Calculating VaR often requires simulating numerous scenarios, making the optimization process computationally intensive, especially for large portfolios.
- Local Optima: Traditional algorithms can get stuck in suboptimal solutions when dealing with VaR, failing to identify the truly best portfolio allocation.
The Future of Portfolio Optimization: Embracing Advanced Algorithms
The BDCA represents a significant step forward in addressing the complexities of VaR-constrained portfolio optimization. Its ability to deliver enhanced performance, faster convergence, and greater reliability makes it a promising tool for financial institutions and investors seeking to navigate today's uncertain market landscape. As computational power continues to grow and algorithmic techniques advance, we can expect even more sophisticated solutions to emerge, further revolutionizing the field of portfolio management.