Decoding Protein Structures: A Guide to Model Evaluation
"Navigating the complexities of protein analysis for groundbreaking advancements in biomedicine and beyond."
Proteins are the workhorses of our cells, and understanding their structure is essential for understanding their function. This knowledge is the bedrock of advancements in biomedicine, drug discovery, and biotechnology. The ability to accurately predict and model protein structures is thus a critical pursuit in modern science.
At the heart of protein structure prediction lies the ability to accurately assess how well a predicted model matches the real thing. Various methods have been developed to tackle this challenge, each with unique strengths and weaknesses. For years, scientists have sought a definitive comparison of these methods to guide their work and improve the reliability of structural predictions.
This article delves into a thorough investigation comparing several popular model assessment methods. It aims to highlight their relative strengths and weaknesses, offering insights for researchers and enthusiasts alike.
Comparative Analysis: Unveiling Evaluation Method Performance
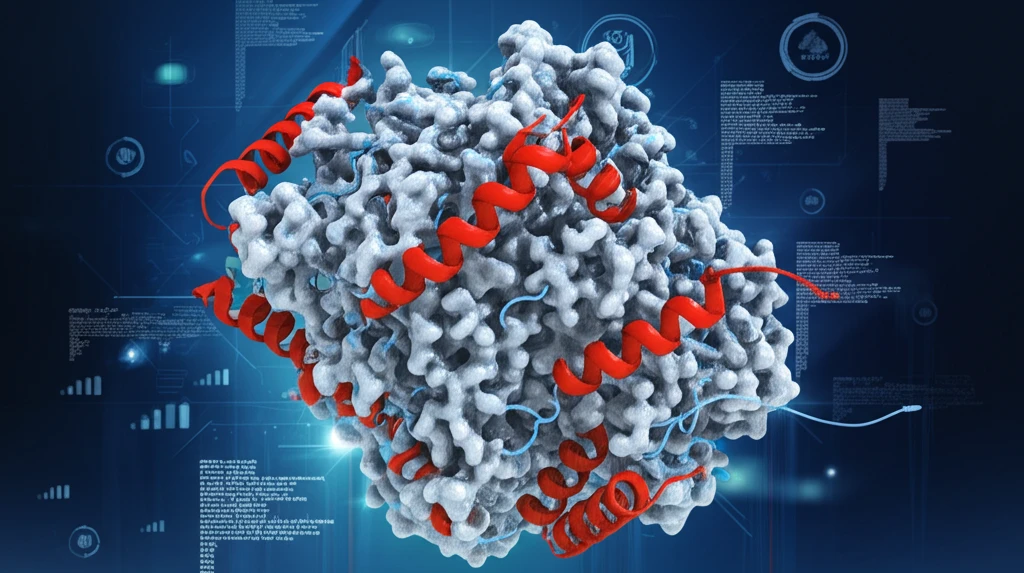
Researchers conducted an extensive analysis of popular protein model assessment methods like RMSD, TM-score, GDT, QCS, CAD-score, LDDT, SphereGrinder, and RPF. This research utilized a diverse set of models from the Community Wide Experiment on the Critical Assessment of Techniques for Protein Structure Prediction (CASP), specifically CASP10-12.
- RMSD: Root Mean Square Deviation
- TM-score: Template Modeling score
- GDT: Global Distance Test
- LDDT: Local Distance Difference Test
Toward Informed Selection and Enhanced Accuracy
This comprehensive comparison offers a valuable resource for researchers navigating the complex landscape of protein structure evaluation. By understanding the strengths and weaknesses of each method, scientists can make informed decisions, leading to more accurate models and groundbreaking advances in the field. Whether developing new prediction tools or refining existing models, the insights from this study provide a solid foundation for future innovation.