Decoding Material Strength: How AI is Revolutionizing Stress Analysis
"A correction in a study highlights the critical role of feature selection methods in stress hotspot classification using AI. Explore the evolving landscape of material science."
In the ever-evolving world of material science, predicting how materials respond to stress is crucial. From designing safer bridges to developing more durable smartphone screens, understanding stress distribution can prevent failures and optimize performance. Recent advancements in artificial intelligence (AI) and machine learning have opened new avenues for analyzing and predicting stress hotspots, areas within a material where stress concentrates and can lead to cracks or breaks.
However, the integration of AI into material science isn't without its challenges. As highlighted by a recent correction to a study published in 'Integrating Materials and Manufacturing Innovation,' the effectiveness of AI models heavily relies on the methods used for feature selection—that is, choosing the most relevant data points to feed into the algorithms. This correction underscores the importance of meticulous methodology and continuous refinement in AI-driven research.
This article delves into the implications of this correction, exploring how AI is transforming stress analysis, the significance of feature selection, and what these advancements mean for industries relying on material durability and safety. With a focus on accessibility and practical insights, we aim to provide a clear understanding of this complex topic for both experts and those new to the field.
The Core of the Correction: What Changed and Why It Matters
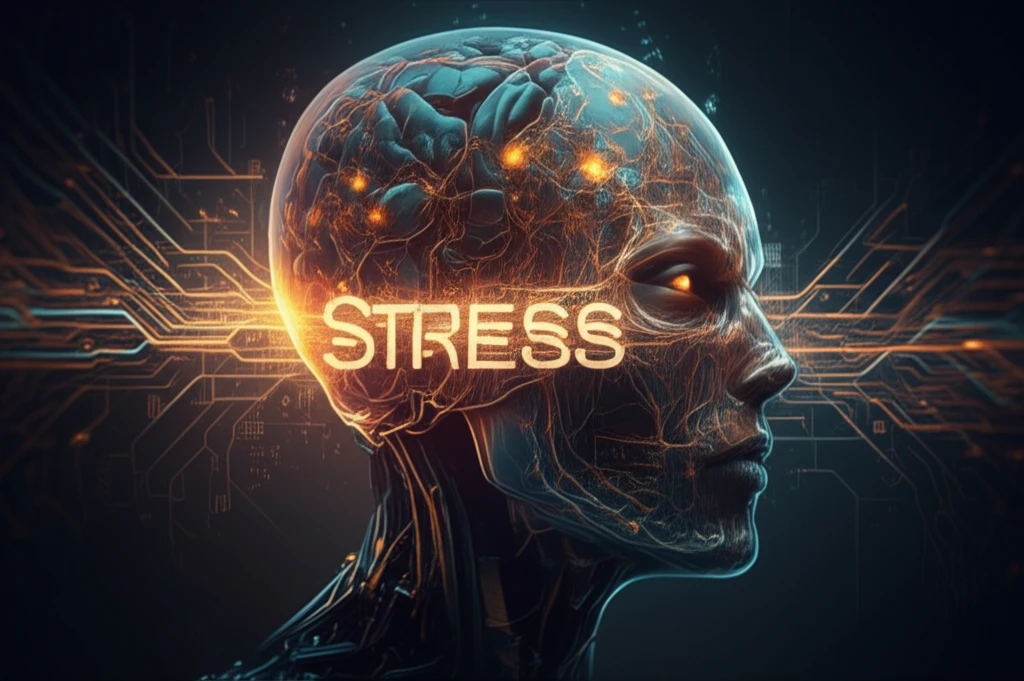
The correction addresses an inconsistency in Table 2 of the original study, where the descriptions of column 1 did not accurately match the text and table footer. This might seem minor, but in scientific research, precision is paramount. The corrected study focuses on comparative feature selection methods for stress hotspot classification, a critical area in materials science. Feature selection involves identifying the most relevant variables or data points that contribute to the accuracy of a predictive model. In this context, it means pinpointing which material characteristics and stress indicators are most important for AI to accurately predict where stress will concentrate.
- Enhanced Accuracy: Correcting discrepancies ensures that the AI models are trained on the most accurate and relevant data.
- Improved Reliability: More precise feature selection leads to more reliable predictions of stress hotspots.
- Better Material Design: Accurate stress analysis informs the design of stronger, more durable materials.
- Reduced Risk of Failure: By identifying potential stress points early, engineers can prevent material failures and ensure structural integrity.
Looking Ahead: The Future of AI in Material Stress Analysis
The correction in this study serves as a reminder of the iterative nature of scientific progress. As AI continues to integrate into material science, ongoing vigilance and refinement will be essential to ensure the accuracy and reliability of these technologies. The future of material stress analysis will likely involve even more sophisticated AI models, capable of handling complex data sets and providing increasingly precise predictions. By prioritizing methodological rigor and data integrity, we can unlock the full potential of AI to create safer, more durable, and more efficient materials for a wide range of applications.