Decoding Market Risk: How Autocorrelations Impact Your Investments
"Understand the Hidden Patterns in Market Price Risks and How They Can Affect Your Portfolio"
In the world of finance, accurately gauging risk is paramount. Whether it's for stringent regulatory compliance, strategic risk management, or ensuring you have enough capital to weather any storm, understanding potential market risks is critical. A key tool in this process is the Value at Risk (VaR), a statistical measure widely used to quantify the potential loss in value of an asset or portfolio over a specific time period.
Many financial institutions rely on methods like Historical Simulation (HS) or the Variance-Covariance approach (often referred to as the 'Wurzel-t-Regel' in German) to calculate VaR. Both methods heavily depend on historical data, drawing insights from past market behavior to predict future risks. However, a significant challenge arises from the inherent dependencies within this historical data, known as autocorrelations. These autocorrelations can subtly yet significantly impact the accuracy of VaR calculations.
This article explores how these autocorrelations affect the measurement of market price risks, providing insights into how they can lead to inaccurate VaR estimates and, consequently, flawed risk management decisions. We will discuss the implications of autocorrelations and offer strategies to mitigate their impact, helping you make more informed and robust investment decisions.
What are Autocorrelations and Why Do They Matter in Risk Measurement?
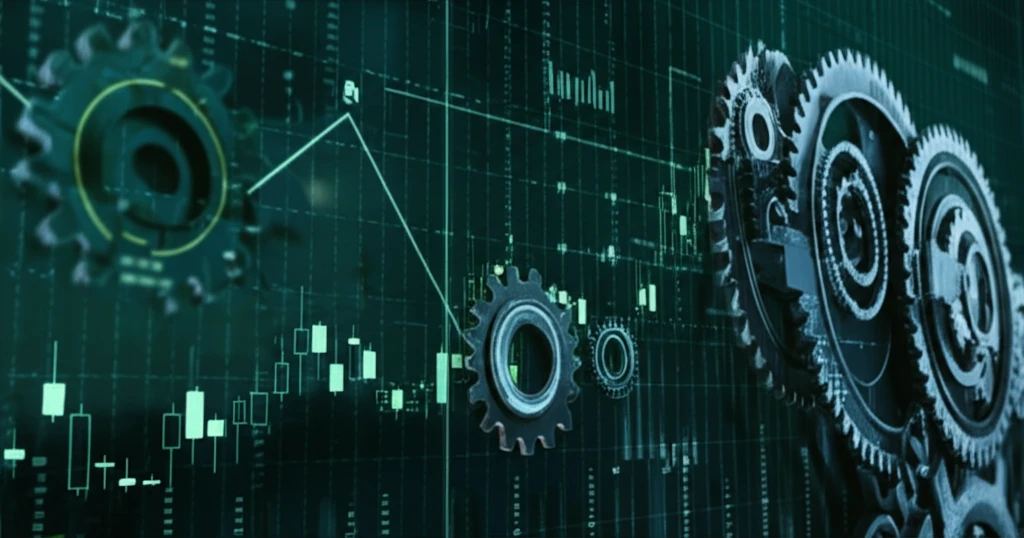
In statistics, correlation quantifies the linear relationship between two different metrics. When this relationship occurs within a single time series—meaning the same metric is observed at different points in time, such as the daily returns of a stock—it's called autocorrelation. Simply put, autocorrelation measures how much past values of a time series influence its future values. If today's stock return is highly dependent on yesterday's return, that series exhibits strong autocorrelation.
- Autocorrelation in Underlying Assets: The returns of market assets, like stocks, often exhibit autocorrelation. Empirical studies have confirmed autocorrelations in the mean and volatility of historical daily returns in both stock and bond markets. While the autocorrelation in the mean is often minimal, its presence signifies that today's return is not entirely independent of yesterday's.
- Autocorrelation from Overlapping Time Windows: When estimating VaR over a long horizon (e.g., a year), analysts frequently use overlapping time windows due to data limitations or relevance concerns. This practice introduces another layer of autocorrelation. Unlike the autocorrelation in daily returns, this is not an economic effect but a statistical artifact of the estimation method.
- Autocorrelation in Backtesting: Backtesting, the process of validating VaR models, can also suffer from autocorrelation issues. For instance, the Kupiec test, a standard backtesting method, assumes that VaR exceedances (when losses exceed the VaR threshold) are independent. However, with overlapping time windows, these exceedances tend to cluster, violating the independence assumption and complicating model validation.
Navigating Autocorrelation for Robust Risk Management
Understanding and addressing autocorrelation is crucial for accurate risk management and informed investment decisions. By recognizing the sources and implications of autocorrelation, investors and financial institutions can refine their risk assessment techniques, leading to more reliable VaR estimates and better capital allocation strategies. Employing methods that account for or mitigate autocorrelation ensures that risk models reflect a more realistic view of market dynamics, ultimately contributing to more stable and resilient financial portfolios.