Decoding Market Instability: Can Quantile Granger Causality Predict Economic Shifts?
"New research offers a robust framework for understanding how economic variables influence each other, even when the markets are turbulent."
In the world of economics, understanding how different factors influence each other is crucial. The concept of Granger causality, developed in 1969, helps economists determine if one time series can predict another. Simply put, if variable X helps predict variable Y, then X "Granger-causes" Y. This concept is vital for investors, policymakers, and anyone trying to make sense of market movements.
Traditional methods often focus on average relationships, which might overlook important connections that occur in the extremes, or “tails,” of the distribution. More recent research has expanded into quantile Granger causality, which examines how variables influence each other across different quantiles (or levels) of the data. This allows for a more nuanced understanding, especially when relationships aren't consistent across the board.
A significant challenge remains: economic relationships aren’t always stable. Market conditions change, policies shift, and unexpected events occur. All these instabilities can throw off traditional causality tests. New research addresses this issue, offering a way to assess Granger causality in quantiles, even when the economic environment is unstable.
Why Standard Granger Causality Tests Fall Short in Unstable Markets
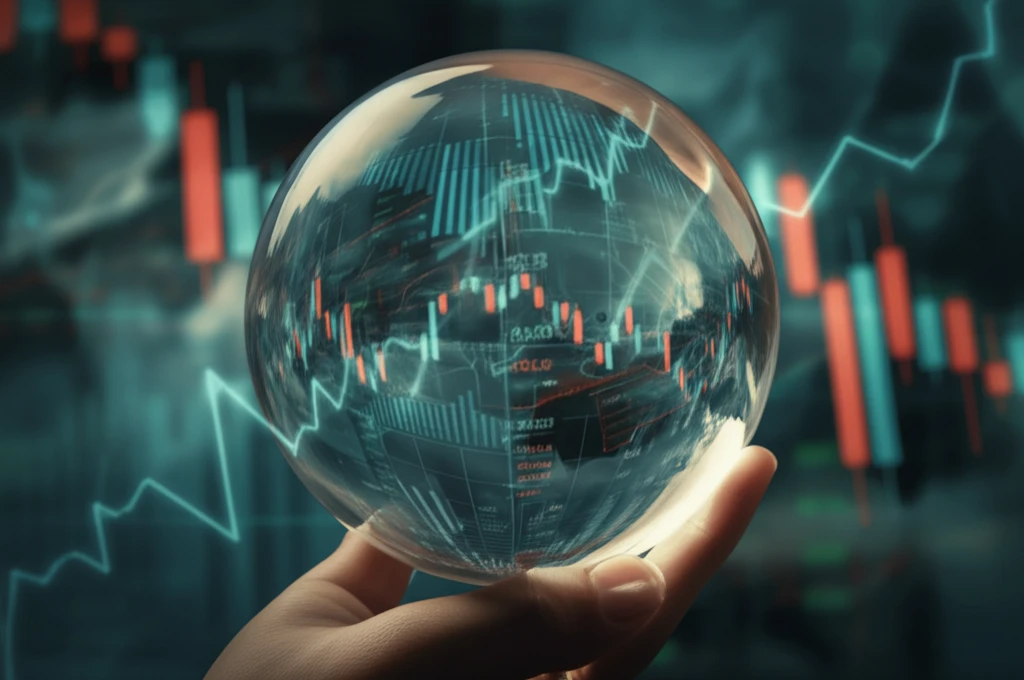
Standard Granger causality tests often assume that relationships between economic variables are constant over time. This simply isn't true in real-world markets, which are constantly affected by fluctuations, volatility, and unforeseen events. Several studies have highlighted how these instabilities can distort the results of traditional causality tests, leading to inaccurate conclusions.
- Fluctuations & Volatility: Financial and macroeconomic data are rarely stable, exhibiting significant volatility.
- Parameter Instabilities: The parameters within economic models can change over time, affecting test performance.
- Structural Breaks: Sudden shifts in economic relationships require methods that can adapt to these changes.
The Future of Economic Forecasting: Robust Causality Testing
The newly developed tests offer a significant advancement in how we understand economic relationships. By accounting for instability and utilizing quantile analysis, these methods provide a more robust and nuanced understanding of Granger causality. This research paves the way for improved economic forecasting, better-informed policy decisions, and more effective investment strategies. As markets continue to evolve and face new challenges, these advanced techniques will become essential tools for navigating the complexities of the global economy.