Decoding Market Dynamics: How State Space Models Unveil Hidden Economic Trends
"Navigate the complexities of economic forecasting with state space models and learn how these models uncover hidden patterns and stochastic process in financial markets"
In today's fast-paced world, understanding the forces that drive financial markets has become more critical than ever. However, the financial sector is subject to constant noise and measurement errors, making it difficult to find underlying patterns. State space models (SSMs) provide a powerful toolkit for analyzing these stochastic processes. These models can extract meaning from complex, error-prone data by focusing on indirect observations of unobservable dynamic processes.
State space models offer a versatile framework for analyzing data, particularly when direct measurements are obscured by noise. SSMs' usefulness lies in their ability to translate complex systems into manageable and interpretable components, whether used to track aircraft on radar or to decode cellphone signals. These models are now essential for specialists looking for deeper insights into hidden market dynamics because of their capacity to manage measurement errors and reveal underlying trends.
This article explores the fundamentals of state space models and how they apply to financial analysis. We will also discuss the statistical underpinnings of SSMs, how to build these models, and how they may be used to explain the dynamics of financial markets. This study aims to equip both seasoned experts and beginners with the information required to use SSMs to enhance their knowledge of economic trends.
State Space Models: A Clearer View of Financial Behaviors
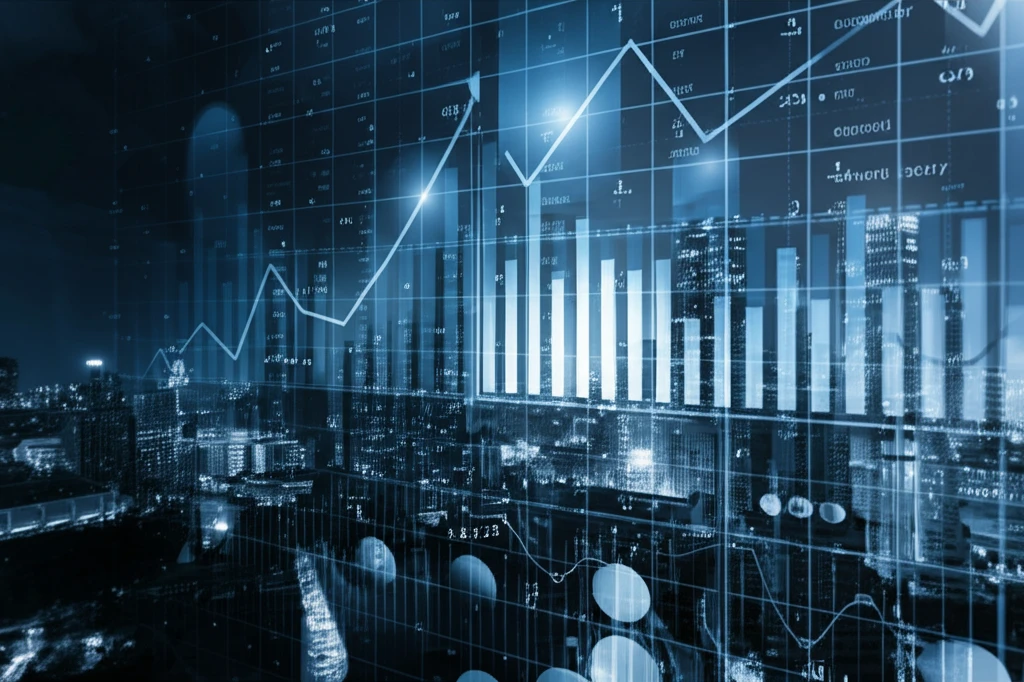
State space models offer a flexible method for analyzing time series data, particularly when dealing with unobserved variables and measurement errors. The heart of an SSM lies in two key equations: the state equation and the observation equation. The state equation describes how the unobserved state variable evolves over time, while the observation equation relates the observed data to the underlying state. SSMs, in contrast to simpler models, can capture complex dependencies and develop through time.
Embracing the Power of SSMs
State space models offer a critical framework for assessing noisy data and identifying underlying patterns in a world where financial markets are becoming more complex. Financial analysts, economists, and data scientists may gain significant insights into market dynamics and improve their forecasting abilities by using state space models. As financial data becomes more complex, SSMs will likely play a key role in assisting experts and academics in navigating the complexities of the global economy.