Decoding High-Dimensional Data: How Estimator Augmentation is Revolutionizing Group Inference
"Unlock insights from complex data sets: A breakthrough in statistical inference offers a more efficient way to analyze groups of parameters."
In today's data-rich world, the ability to extract meaningful insights from high-dimensional data is more critical than ever. From genomics to social sciences, vast datasets present both opportunities and challenges. Statistical inference, the process of drawing conclusions from data, plays a vital role, but traditional methods often struggle with the complexities of high-dimensional models.
One common task is group inference, where the goal is to understand the relationships among a group of parameters. Think of identifying a set of genes that collectively influence a disease or a cluster of economic indicators that drive market trends. These types of analyses are notoriously difficult, especially when the number of potential predictors far exceeds the number of observations.
Now, a groundbreaking approach called estimator augmentation offers a powerful new way to tackle these challenges. This method significantly enhances the efficiency and accuracy of group inference, paving the way for more reliable insights from complex datasets.
What is Estimator Augmentation and Why Does It Matter?
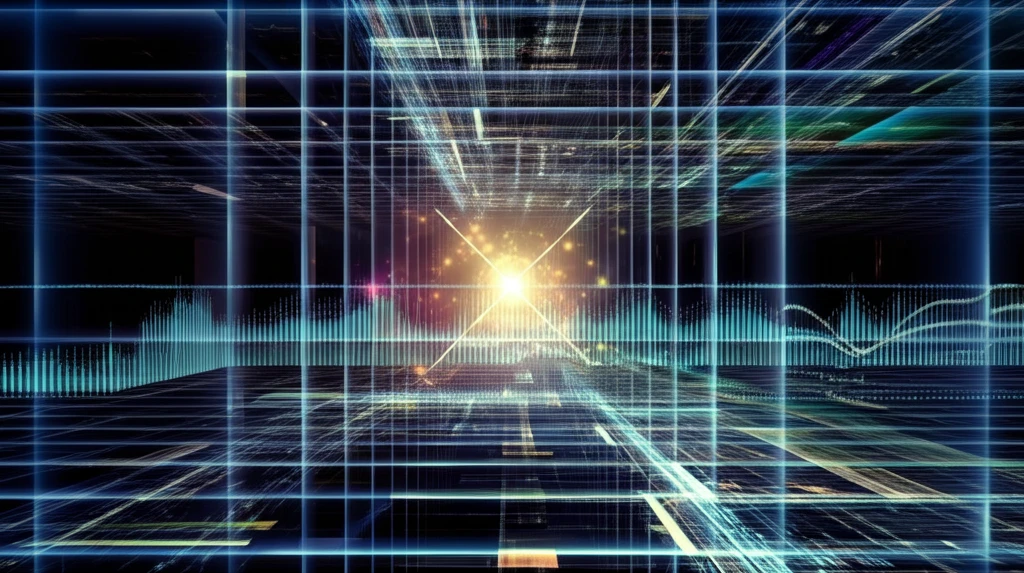
Estimator augmentation is a statistical technique designed to improve inference about groups of parameters in high-dimensional settings. It focuses on refining existing estimators, like the block lasso, which uses regularization to handle large numbers of variables. The method works by adding extra information, or "augmentation," to the estimator, allowing for a more precise understanding of its behavior.
- Increased Efficiency: Estimator augmentation can be orders of magnitude more efficient than traditional methods like bootstrapping, especially when estimating tail probabilities for significance tests.
- Improved Accuracy: By providing a more accurate picture of the estimator's sampling distribution, this method leads to more reliable conclusions.
- New Insights: Estimator augmentation sheds light on the geometry of the sample space and the uniqueness of solutions, providing a deeper understanding of the underlying data structure.
The Future of Data Analysis is Here
Estimator augmentation represents a significant step forward in our ability to analyze and interpret complex, high-dimensional data. By providing a more efficient and accurate approach to group inference, this method empowers researchers and analysts to unlock valuable insights across a wide range of fields. As data continues to grow in both size and complexity, techniques like estimator augmentation will be essential for making sense of the world around us.