Decoding High-Dimensional Data: How Estimator Augmentation Can Revolutionize Group Inference
"Unlock the power of statistical inference with estimator augmentation, a new method for analyzing complex data and making accurate group inferences."
In today's data-rich world, high-dimensional data is becoming increasingly prevalent across various fields, from genomics and finance to social sciences and marketing. This type of data presents unique challenges for statistical inference, as traditional methods often struggle to handle the complexity and dimensionality. As a result, researchers and practitioners are constantly seeking new and innovative techniques to extract meaningful insights from these complex datasets.
Statistical inference for high-dimensional data is crucial for identifying significant patterns and relationships, and for making informed decisions based on the data. One common task is group inference, which involves making statements about a group of parameters rather than individual ones. However, performing group inference on high-dimensional data is particularly challenging due to the large number of parameters and the potential for complex dependencies between them.
To address these challenges, a novel method called estimator augmentation has emerged as a promising approach. Estimator augmentation enhances existing estimators, such as the block lasso, by incorporating additional information about the structure of the data. This approach can lead to more efficient and accurate statistical inference, particularly for group inference problems. This article explores the principles behind estimator augmentation, its applications in high-dimensional group inference, and its advantages over traditional methods.
What is Estimator Augmentation and How Does It Work?
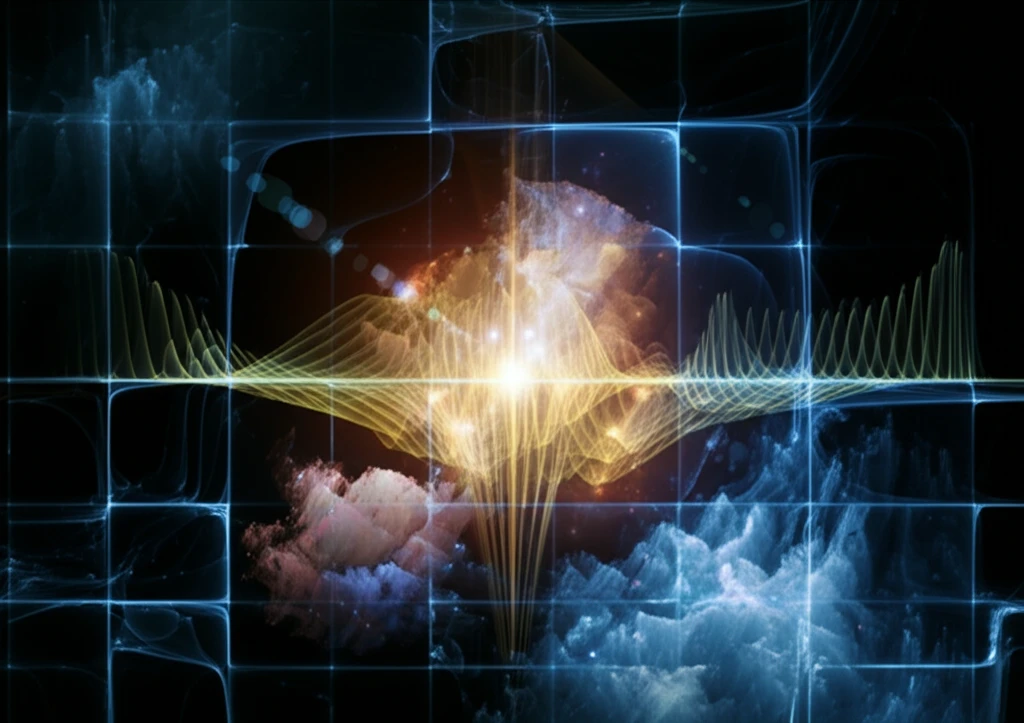
Estimator augmentation is a statistical technique designed to improve the performance of estimators in high-dimensional settings. It works by incorporating additional information about the data, such as the subgradient of a regularization term, into the estimation process. In the context of the block lasso, a popular method for group inference, estimator augmentation involves augmenting the block lasso estimator with the subgradient of the block norm.
- Improved Accuracy: By incorporating additional information, estimator augmentation can lead to more accurate estimates of the parameters of interest.
- Enhanced Efficiency: The closed-form density allows for the use of Monte Carlo methods, which can be more efficient than traditional bootstrap techniques.
- Greater Flexibility: Estimator augmentation can be applied to a wide range of estimators and regularization techniques.
- Better Understanding of Uncertainty: The joint distribution of the estimator and its subgradient provides a more complete picture of the uncertainty associated with the estimates.
The Future of Statistical Inference with Estimator Augmentation
Estimator augmentation is a significant advancement in the field of high-dimensional statistics, offering a powerful and efficient approach to group inference. By combining estimator augmentation with Monte Carlo methods, researchers and practitioners can overcome the limitations of traditional techniques and gain deeper insights from complex datasets. As the volume and dimensionality of data continue to grow, estimator augmentation is poised to play an increasingly important role in statistical inference and data analysis.