Decoding Hidden Patterns: How Bivariate Hidden Markov Models Are Revolutionizing Insurance Claim Prediction
"Unlock the secrets of claim dependence: A guide to using advanced statistical methods for smarter insurance risk management."
For insurance companies, accurately predicting claims is crucial for maintaining financial health and ensuring policyholders are protected. Traditionally, insurers have relied on various statistical models to estimate claim frequencies and amounts. However, these models often assume independence between different claims, which can be a simplification that doesn't reflect real-world complexities.
In reality, claims are rarely isolated events. Economic conditions, weather patterns, and even social trends can influence both the number of claims and the size of payouts. Ignoring these dependencies can lead to inaccurate predictions and, ultimately, financial instability for insurance providers.
Enter the Bivariate Hidden Markov Model (BHMM), a sophisticated statistical tool designed to capture these hidden dependencies. By analyzing claim numbers and aggregate claim amounts together, BHMMs can uncover underlying patterns and provide more accurate forecasts, helping insurers make informed decisions and manage risk effectively.
What Are Bivariate Hidden Markov Models (BHMMs)?
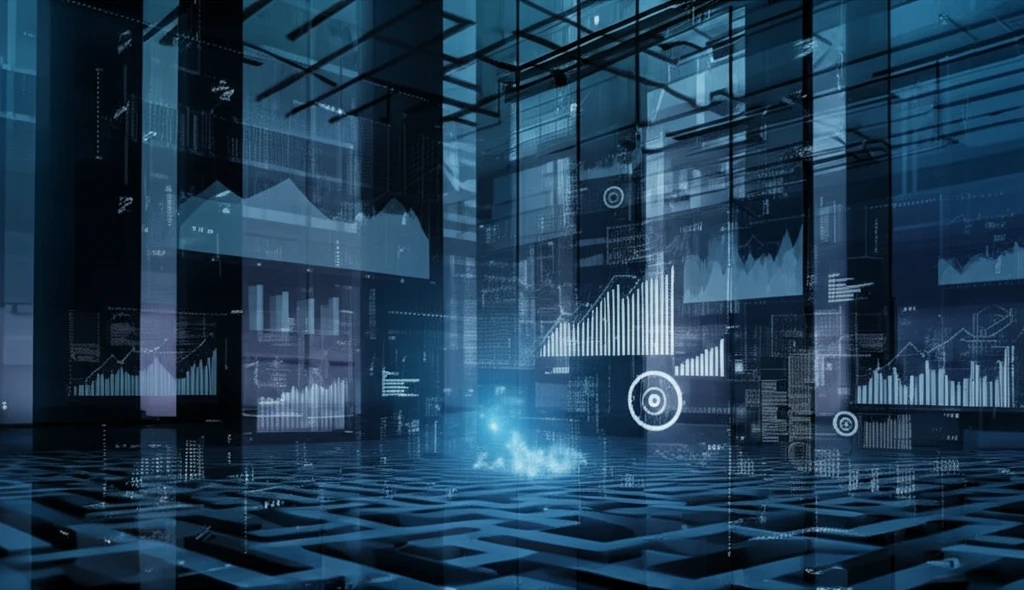
At its core, a BHMM is a statistical model that assumes there are underlying, unobservable 'states' that influence the observed claim data. These hidden states could represent various factors, such as economic conditions (e.g., recession, growth), seasonal variations (e.g., winter, summer), or even shifts in consumer behavior. The model analyzes both the number of claims and the total amount of claims to infer which hidden state is most likely at any given time.
- State-Dependent Distributions: BHMMs use different probability distributions for each hidden state to model the claim numbers and amounts. Common distributions include Poisson, Normal, Gamma, and Negative Binomial.
- Expectation Maximization (EM) Algorithm: This algorithm is used to estimate the parameters of the BHMM, including the probabilities of transitioning between hidden states and the parameters of the state-dependent distributions.
- Viterbi Algorithm: Once the model is trained, the Viterbi algorithm can be used to determine the most likely sequence of hidden states given a set of observed claim data.
The Future of Insurance Claim Prediction
Bivariate Hidden Markov Models represent a significant advancement in insurance claim prediction. By embracing the complexities of claim dependencies and leveraging sophisticated statistical techniques, insurers can gain a more accurate understanding of their risk exposure. As the insurance landscape continues to evolve, BHMMs offer a powerful tool for navigating uncertainty and ensuring financial stability. While the implementation and interpretation of BHMMs require specialized expertise, the potential benefits for insurers are undeniable. Embracing these advanced techniques is a step toward a more data-driven and resilient future for the insurance industry.