Decoding Hidden Biases: How Causal Machine Learning Can Lead to Fairer Decisions
"Uncover the secrets of omitted variable bias and learn how new techniques in causal machine learning are helping to build more reliable and equitable AI systems."
In an era increasingly shaped by algorithms, the promise of objective decision-making through artificial intelligence is often undermined by a pervasive issue: bias. While machine learning models excel at identifying patterns, they can inadvertently amplify existing societal inequalities if trained on biased data or if crucial factors are overlooked. This article delves into the challenges of omitted variable bias in causal machine learning and explores cutting-edge techniques designed to create more equitable and reliable AI systems.
Omitted variable bias occurs when a statistical model leaves out one or more relevant variables, leading to skewed or inaccurate conclusions. Imagine, for example, a loan application model that doesn't consider historical discrimination in housing. Such a model might unfairly deny loans to applicants from certain neighborhoods, perpetuating existing inequalities. Recognizing and addressing these biases is crucial for building AI that serves everyone fairly.
Fortunately, researchers are developing innovative methods to tackle omitted variable bias head-on. This article will unpack a general theory of omitted variable bias in causal machine learning, revealing how simple adjustments can lead to more robust and equitable outcomes. We'll explore real-world applications, offering insights into how these techniques can be implemented across various sectors to ensure AI benefits all members of society.
What is Omitted Variable Bias and Why Does It Matter?
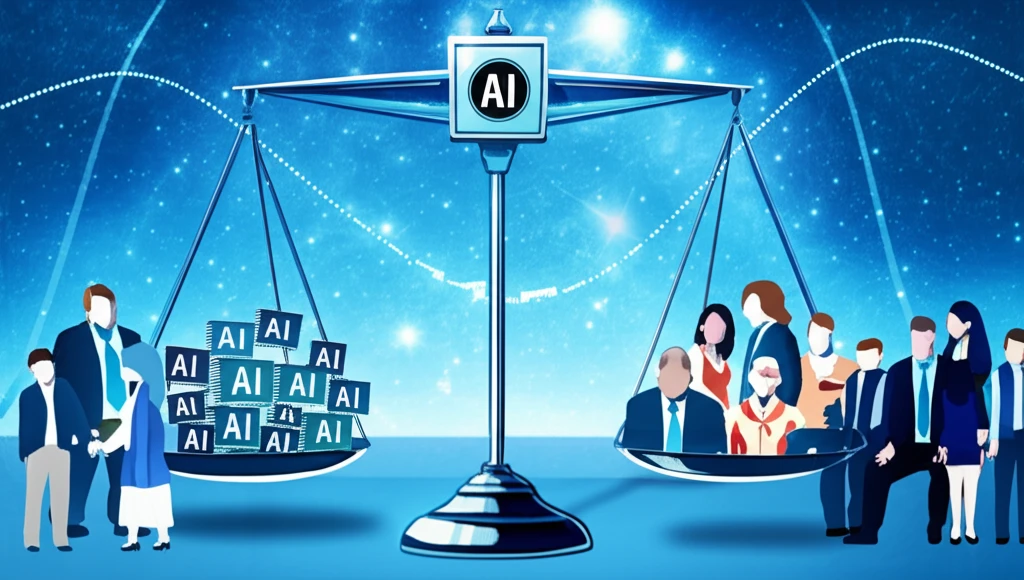
Omitted variable bias (OVB) is a critical issue in causal inference, arising when relevant variables are left out of a statistical model. This can lead to distorted estimates of causal effects, making it difficult to understand the true relationships between variables. In the context of machine learning, OVB can result in biased predictions and unfair outcomes, particularly when dealing with complex, nonlinear models.
- Impacts of OVB: Skewed or inaccurate conclusions.
- Relevance: Understanding true relationships between variables.
- Real-world consequences: Biased predictions and unfair outcomes.
The Future of Fairer AI
As AI systems become more deeply integrated into our lives, the need for robust and equitable models will only intensify. The techniques discussed in this article represent a significant step forward in addressing the challenge of omitted variable bias and building AI that truly benefits all members of society. By embracing causal machine learning and prioritizing fairness, we can unlock the full potential of AI while mitigating its risks, creating a future where technology promotes equality and opportunity for everyone.