Decoding Financial Time Series: Can Fuzzy Models Predict the Market?
"Explore how fuzzy logic and AR models are reshaping financial forecasting."
In the world of predicting how financial markets will behave, experts are always looking for better tools. For a long time, they've leaned on methods from math and stats to try and make sense of the ups and downs in market data. These methods have been really important, especially in processing signals that change over time. They've been used a lot in areas like figuring out the best ways to control things and understanding how the economy works.
One big step forward was using linear models, which came from the work of Box and Jenkins. But as time went on, people in economics started using other types of models too. These new models could handle more complex stuff, like when things don't follow a straight line, using tools like TAR, STAR, and ARCH models.
More recently, with the rise of artificial intelligence, new techniques like fuzzy models and neuro-fuzzy systems are being used to analyze and predict time series data. These methods, part of what's called Computational Intelligence, have been around for a few decades, but we still need to compare them closely with the older methods. It's important to know which tools work best, how accurate they are, and how easy they are to use. This article explores how these AI-driven fuzzy models stack up against traditional autoregressive models in the world of financial forecasting.
AR Models vs. Fuzzy Models: A Deep Dive
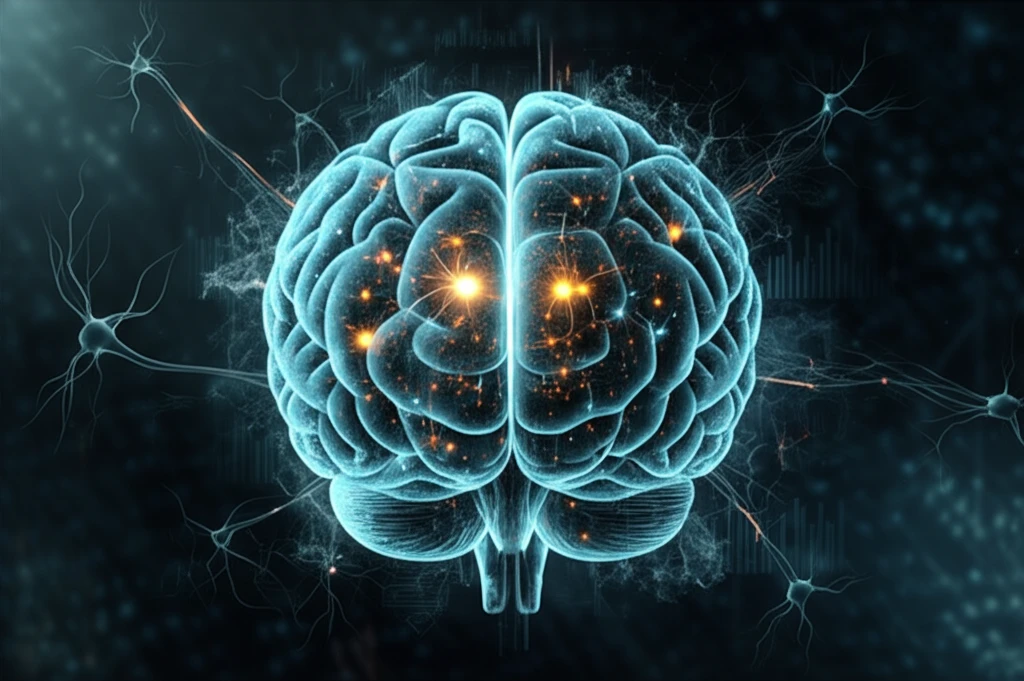
At the heart of this analysis is a comparison between two main types of models: autoregressive (AR) models and fuzzy models. AR models work by assuming that future values in a time series can be predicted from a linear mix of past values. Think of it like predicting tomorrow's stock price based on a combination of the prices from the last few days. These models are defined by a few key things: a white noise process (random fluctuations), a finite variance (how spread out the data is), and a covariance function (how the data points relate to each other).
- State-Space Granulation: How each model divides the data into manageable chunks.
- Mathematical Form: The actual equations used by AR models and fuzzy models (Mamdani, TSK).
- Statistical Metrics: Measures like Mean Squared Error (MSE) and autocorrelation of residuals to check accuracy.
The Future of Financial Forecasting
In summary, both AR models and fuzzy models offer unique ways to tackle financial time series. AR models are straightforward and work well when the data is stable. Fuzzy models, on the other hand, can handle more complex situations but require careful setup. The best approach depends on the specific problem and the characteristics of the data. As computational methods continue to advance, combining these techniques may lead to even more accurate and reliable financial forecasts.