Decoding Financial Flows: Can Topology Unlock Macro Trading Opportunities?
"Move beyond traditional econometrics and discover how stochastic flow diagrams can illuminate the complex world of financial markets."
In today's interconnected global economy, financial markets are in constant motion. Capital flows ebb and surge across asset classes, industries, and geographical boundaries, creating both opportunities and risks. Understanding these flows is critical for investors, policymakers, and regulators alike. However, traditional methods of financial analysis often fall short, struggling to capture the complex, dynamic relationships that drive market behavior.
A groundbreaking study published in Algorithmic Finance proposes a novel approach: using topological methods and Stochastic Flow Diagrams (SFDs) to map and analyze macro financial flows. This innovative framework moves beyond traditional econometrics, which often focuses on static relationships and linear models, to embrace the dynamic, interconnected nature of financial systems.
By visualizing capital flows as networks and applying tools from graph theory, researchers can gain new insights into how shocks propagate through the financial system, identify key vulnerabilities, and potentially uncover hidden trading opportunities. This approach offers a powerful complement to existing analytical techniques, promising to enhance our understanding of market dynamics and improve decision-making in an increasingly complex world.
Why Traditional Financial Models Fall Short?
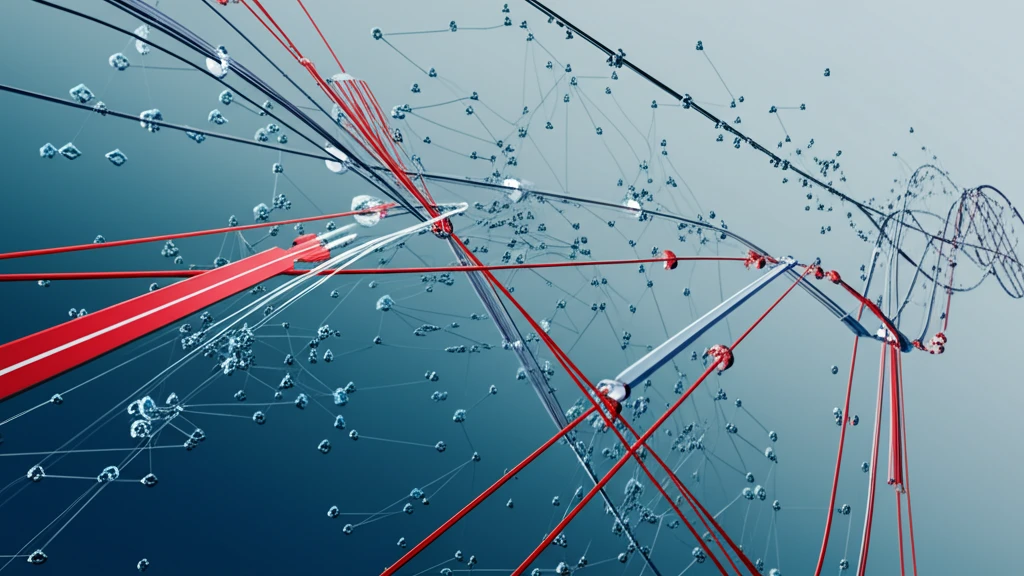
Classical financial research relies heavily on linear algebra such as regression analysis, and Stochastic Calculus such as valuation models. The limitation is that both approaches primarily focus on geometric locations rather than the more crucial logical relations between system components.
- Static vs. Dynamic: Traditional correlation analysis offers a snapshot in time, failing to capture the evolving nature of financial relationships.
- Causation vs. Correlation: Correlation does not imply causation, and traditional methods often struggle to identify true cause-and-effect relationships.
- Linearity Assumption: Financial systems are inherently non-linear, and linear models may oversimplify complex interactions.
- Lack of Interconnectedness: Traditional models often treat markets in isolation, neglecting the crucial links that tie them together.
The Future of Financial Analysis
As financial markets become increasingly complex and interconnected, the need for more sophisticated analytical tools will only grow. Topological methods and SFDs offer a promising path forward, providing a framework for understanding the dynamic relationships that drive market behavior. By embracing these innovative approaches, investors, policymakers, and regulators can gain a deeper understanding of the financial system and make more informed decisions in an ever-changing world.