Decoding Economic Mysteries: How Predictable Errors Can Revolutionize Forecasting
"Uncover the hidden power of predictable errors in economic forecasting and learn how new imputation methods can lead to more accurate outcomes."
In the world of economics, making accurate predictions is essential for governments, businesses, and individuals alike. Whether it’s forecasting GDP growth, anticipating market trends, or evaluating the impact of policy changes, reliable predictions can drive better decisions and more stable outcomes. But what happens when the models we rely on aren’t perfect? What if there are predictable errors lurking beneath the surface, distorting our forecasts and leading us astray?
Traditional economic models often focus on sampling uncertainty, which assumes that errors will diminish as the sample size grows. However, a less explored but equally important source of error lies in the predictability of out-of-sample information. This type of error occurs when information not captured by the model can actually inform us about the missing counterfactual outcome, especially if these errors are correlated over time or across different economic entities.
Imagine trying to predict the economic impact of a new policy. You might build a model based on historical data and various economic indicators. But what if the model doesn’t fully account for certain factors, leading to errors in its predictions? If these errors are random, they might average out over time. However, if they are predictable – perhaps influenced by factors outside the model – ignoring them could lead to significantly skewed results. This is where new approaches to economic forecasting come into play, promising to turn these predictable errors into opportunities for improvement.
The Problem with Overlooking Predictable Errors
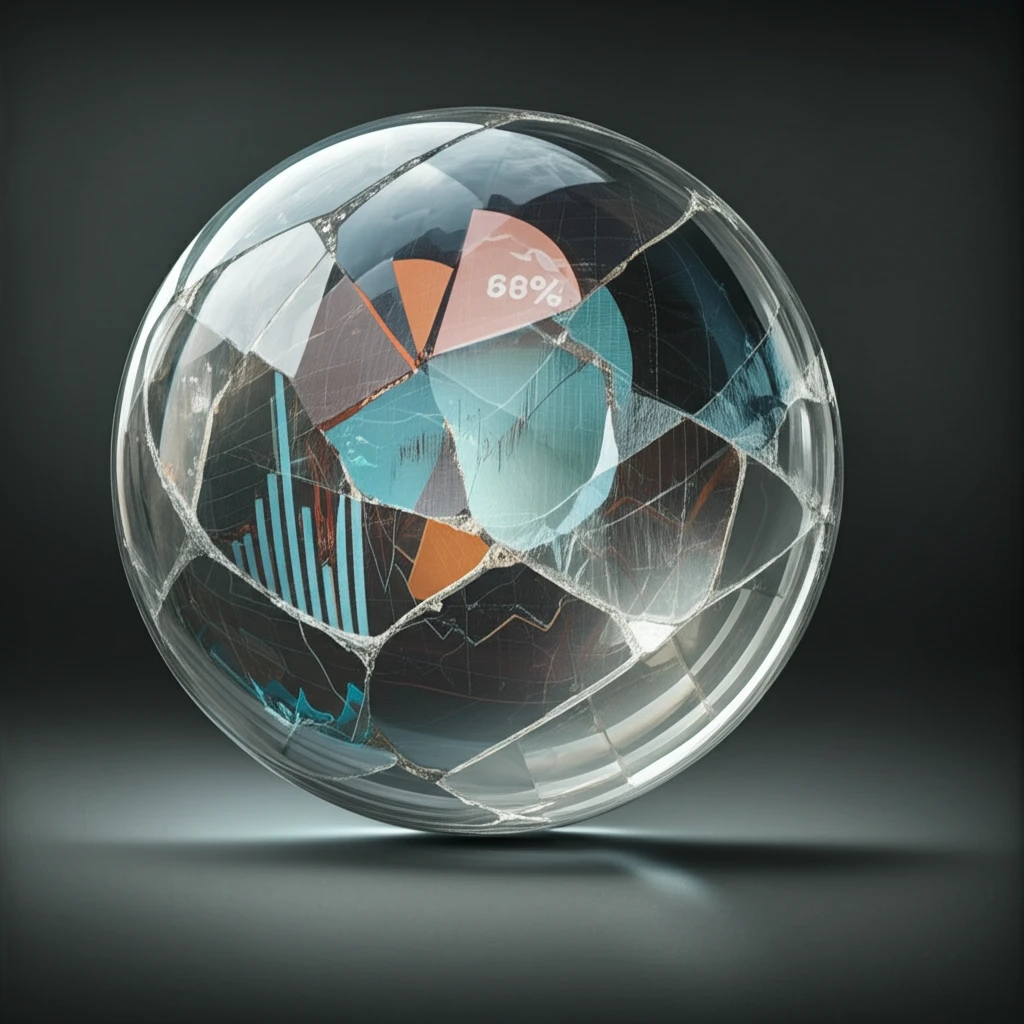
The standard approach to economic modeling often overlooks the potential for errors to be predictable. Traditional methods assume that as the sample size increases, the uncertainty in the model will decrease, leading to more accurate predictions. However, this assumption doesn't hold when out-of-sample errors contain valuable information about the missing counterfactual outcome.
- Mis-specification: Errors can arise if the model doesn't accurately represent the underlying economic relationships. For instance, assuming a simple linear relationship when the true relationship is more complex.
- Incomplete Information: Models often can't capture all the relevant information, leading to errors that reflect the missing factors. This could include unforeseen events, behavioral changes, or other hard-to-quantify influences.
- Temporal Aggregation: Aggregating data over time can introduce serial correlation in the errors. For example, monthly data might be more volatile than quarterly data, leading to predictable patterns in the errors.
Looking Ahead: Embracing Predictable Errors for Better Economic Insights
The future of economic forecasting lies in acknowledging and harnessing the information contained within predictable errors. By moving beyond traditional methods that assume randomness, economists can unlock new levels of accuracy and gain deeper insights into the complex forces that shape our world. Embracing these new techniques promises to create more robust and reliable economic models, leading to better-informed decisions and a more stable economic future for everyone.