Decoding Economic Models: How to Navigate Endogenous Control Variables
"Unlock clarity in economic analysis. Learn how to handle endogenous control variables and improve your understanding of treatment effects in research."
Economic modeling often involves complex relationships where cause and effect are not always straightforward. One common challenge researchers face is dealing with 'endogenous control variables.' Simply put, these are control variables that are themselves influenced by other factors within the model, leading to biased or misleading results if not handled correctly. It’s like trying to bake a cake when the oven temperature is constantly changing—the outcome becomes unpredictable.
Consider a scenario where you're trying to determine the impact of a job training program on individuals' wages. Ideally, you would want to control for factors like education level or prior work experience. However, what if access to better education is also influenced by family income, which in turn affects participation in the job training program? This creates a loop, making it difficult to isolate the true effect of the training program.
This article breaks down the complexities of endogenous control variables, offering simplified explanations inspired by recent research. Whether you're an economics student, a policy analyst, or just someone curious about how economic models work, you'll gain practical insights into handling these tricky variables and improving the accuracy of your analysis.
What are Endogenous Control Variables and Why Do They Matter?
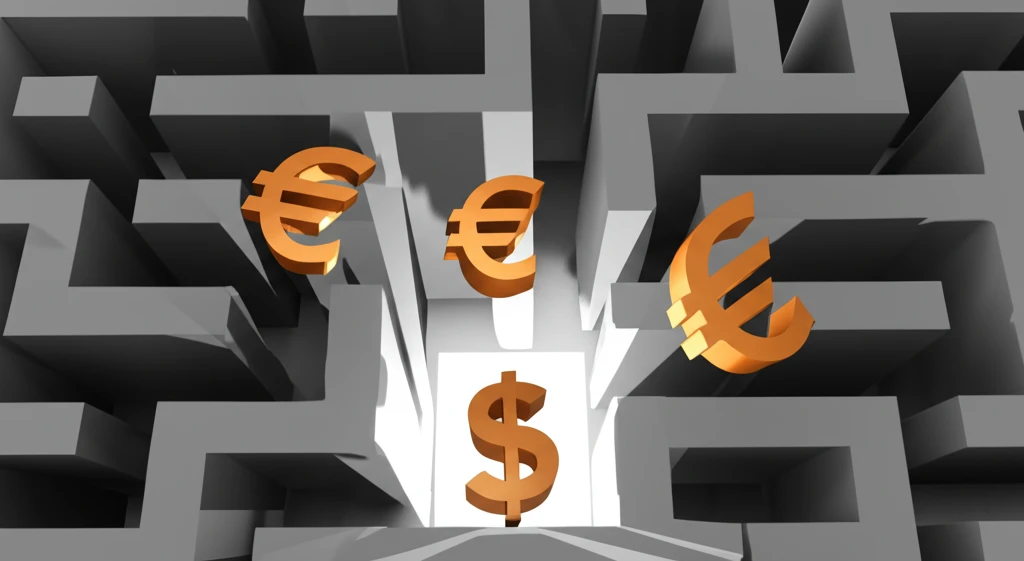
Endogenous control variables create a situation where the traditional methods of assessing treatment effects become unreliable. In simpler terms, imagine you're trying to measure the effect of a new fertilizer on crop yield. You control for factors like sunlight and water, but what if the farmers using the fertilizer also tend to use more advanced irrigation techniques? The irrigation (a control variable) is influenced by the adoption of the fertilizer (the treatment), making it difficult to isolate the fertilizer’s true impact.
- Bias in Estimates: Endogeneity can lead to biased estimates of treatment effects, making it difficult to draw accurate conclusions.
- Incorrect Policy Implications: Flawed analysis can result in ineffective or even harmful policy recommendations.
- Inefficient Resource Allocation: Misunderstanding the true drivers of outcomes can lead to wasted resources and missed opportunities.
- Compromised Business Strategies: Businesses relying on faulty data may make poor decisions about investments and marketing efforts.
Making Better Decisions with Clearer Economic Models
Understanding how to deal with endogenous control variables is essential for anyone working with economic data. By applying these techniques, researchers and analysts can develop more robust models, leading to better-informed decisions and more effective strategies. Embracing these methods not only enhances the accuracy of economic analysis but also fosters a deeper understanding of the complex forces shaping our world.