Decoding Economic Models: How Efficient Tests Can Navigate Non-Regular Scenarios
"Discover how economists are refining testing methods to ensure accuracy and relevance in complex, real-world situations, where traditional tools fall short."
In the intricate world of economics, models serve as vital tools for understanding and predicting market behaviors, guiding policy decisions, and assessing financial risks. These models, however, are not infallible. They are often built on assumptions that, in reality, do not always hold true. One significant challenge arises when dealing with 'non-regular' models, where traditional statistical methods falter due to the violation of standard assumptions.
Traditional economic tests often rely on the principle of 'local regularity,' which assumes that the behavior of estimators—methods used to approximate the values of parameters—remains consistent under small changes to the model’s parameters. However, many economic scenarios defy this regularity. For instance, models dealing with weak identification—situations where the data provides little information about key parameters—or those involving infinite-dimensional nuisance parameters—unnecessary parameters—can render standard tests unreliable.
Recently, economist Adam Lee has introduced novel approaches to tackle these challenges, focusing on developing tests that remain accurate and efficient even in non-regular environments. His work addresses the critical need for robust testing methodologies that can adapt to the complexities of modern economic models, ensuring that policy decisions and market analyses are based on sound, reliable evidence. This article delves into Lee's innovative methods and their implications for economic analysis.
What Makes Economic Models 'Non-Regular'?
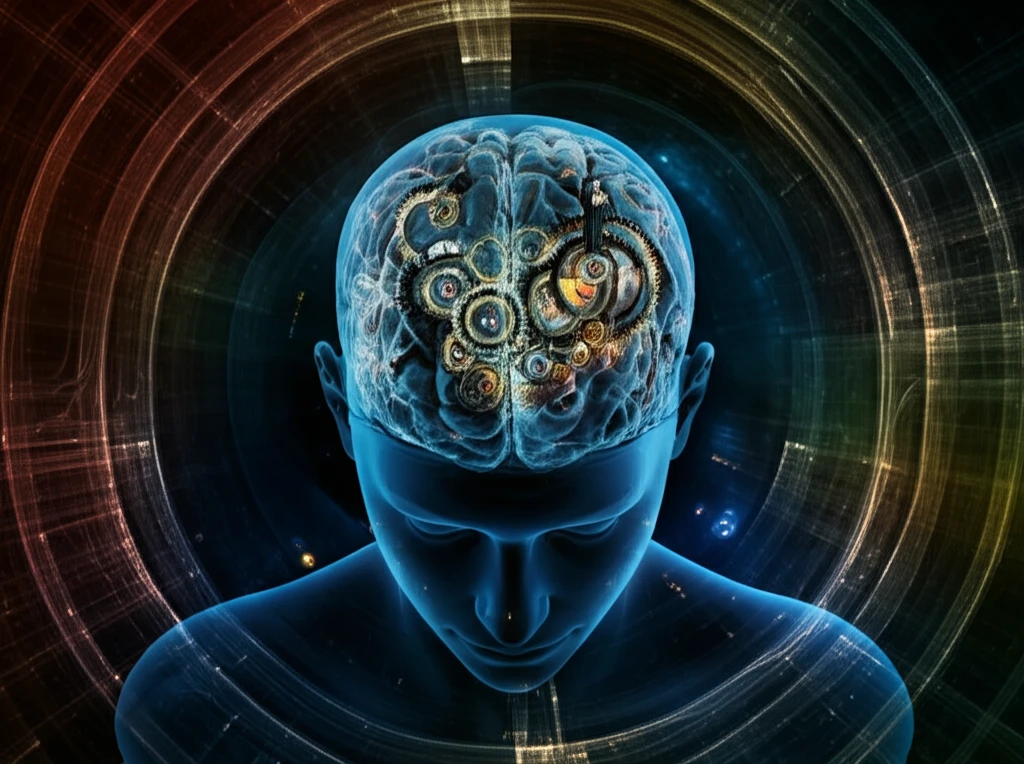
The term 'non-regular' in economic modeling describes scenarios where conventional statistical assumptions are not met, leading to unreliable estimations and tests. This often occurs when dealing with models that exhibit:
- Weak Identification: Situations where the available data offers little insight into the parameters of interest. This can happen when key variables are poorly correlated or when the model is inherently insensitive to changes in certain parameters.
- Infinite-Dimensional Nuisance Parameters: Models that include a large number of unnecessary or irrelevant parameters, complicating the estimation process and potentially distorting results.
- Boundary Issues: Cases where parameters are at or near the boundary of their possible values, violating assumptions of standard statistical tests.
Why This Matters for Economists and Policymakers
The development of locally regular and efficient tests represents a significant advancement in economic methodology. By providing tools that function reliably in non-regular models, Lee's work enhances the accuracy and relevance of economic analysis. This is particularly crucial for policymakers and financial analysts who rely on these models to make informed decisions that can affect markets and economies.