Decoding Economic Forecasts: How Factor-Augmented Sparse MIDAS Regressions Are Changing the Game
"Discover how a new approach to economic forecasting combines the best of sparse and dense data analysis to predict recessions with greater accuracy."
In an era defined by economic uncertainty, the ability to accurately forecast macroeconomic variables is more critical than ever. Policymakers, investors, and businesses rely on these forecasts to make informed decisions, plan for the future, and mitigate risks. Traditional forecasting methods, however, often struggle to handle the high-dimensional, mixed-frequency data that characterizes modern economies. This challenge has spurred the development of innovative techniques designed to extract meaningful signals from complex datasets.
One such innovation is the factor-augmented sparse MIDAS (Mixed Data Sampling) regression, a sophisticated approach that combines the strengths of several dimensionality reduction techniques. This method is designed to handle the complexities of high-dimensional time series data, where variables are observed at different frequencies—think weekly financial data alongside monthly macroeconomic indicators. By integrating sparse and dense dimensionality reduction, factor-augmented sparse MIDAS regressions offer a more nuanced and accurate way to nowcast and forecast economic trends.
This article delves into the mechanics of factor-augmented sparse MIDAS regressions, exploring how they outperform traditional methods, especially during periods of economic turbulence. We'll break down the key concepts, examine real-world applications, and discuss the implications of this technique for economic forecasting.
What Are Factor-Augmented Sparse MIDAS Regressions?
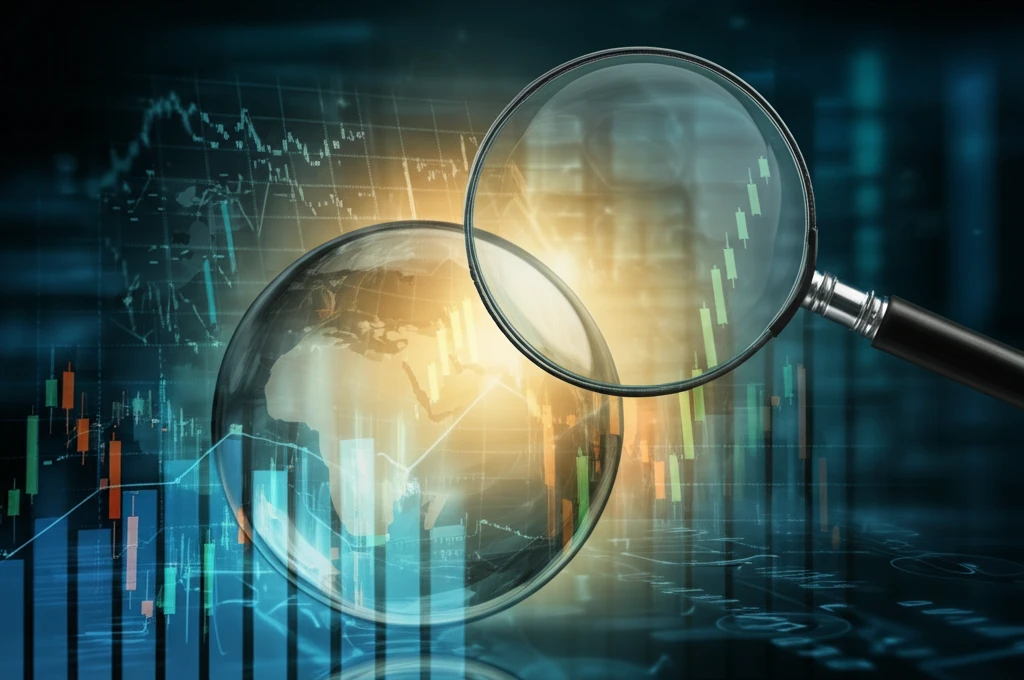
Factor-augmented sparse MIDAS regressions represent a fusion of several powerful statistical techniques, each addressing specific challenges in economic forecasting. To fully grasp this approach, it’s helpful to understand its core components:
- Factor-Augmented Regressions: These techniques, pioneered by Stock and Watson, extract information from a large number of variables by identifying a smaller set of latent factors. This assumes a low-dimensional factor structure within the high-dimensional covariate matrix, effectively reducing the complexity of the data.
- Sparse Methods: In contrast, sparse methods like LASSO regression focus on identifying a small subset of predictors most relevant for forecasting. This approach adheres to the principle of parsimony, emphasizing that only a few key variables significantly influence the outcome.
- Mixed Data Sampling (MIDAS): Economic data often comes in mixed frequencies, such as weekly financial data alongside monthly macroeconomic data. MIDAS regressions address this by applying a weighting scheme to directly link high-frequency data with low-frequency series, mitigating parameter proliferation in high-dimensional lag structures.
The Future of Economic Forecasting
Factor-augmented sparse MIDAS regressions represent a significant step forward in economic forecasting, offering a more nuanced and accurate way to navigate the complexities of modern economies. By combining the strengths of various dimensionality reduction techniques, this approach provides valuable insights for policymakers, investors, and businesses seeking to make informed decisions in an uncertain world.