Decoding Dyadic Data: A Practical Guide to Overcoming Sample Selection Bias in Network Analysis
"Unlock deeper insights from your network data. Learn how to handle sample selection bias, improve model accuracy, and gain a competitive edge."
In today's data-driven world, understanding relationships and interactions is paramount. Dyadic data, which describes pairwise outcomes such as trade between countries or migration patterns, offers valuable insights into these connections. However, dyadic data often presents a significant challenge: sample selection bias. This bias arises when the observed data is not a random sample of all possible pairs, leading to skewed results and inaccurate conclusions.
Imagine analyzing migration flows between states, but only considering pairs where migration actually occurs. This ignores the many state-pairs with no migration, potentially distorting your understanding of the factors that drive movement. Similarly, in trade analysis, neglecting country-pairs with no trade can lead to flawed conclusions about trade agreements and economic policies. Addressing this bias is crucial for reliable and actionable insights.
This article provides a practical guide to understanding and overcoming sample selection bias in dyadic data analysis. We'll explore the causes of this bias, introduce effective techniques for mitigating its effects, and demonstrate how these methods can enhance the accuracy and robustness of your findings. Whether you're a researcher, data scientist, or business analyst, this guide will equip you with the tools to unlock the full potential of your network data.
What is Dyadic Data and Why Does Sample Selection Bias Matter?
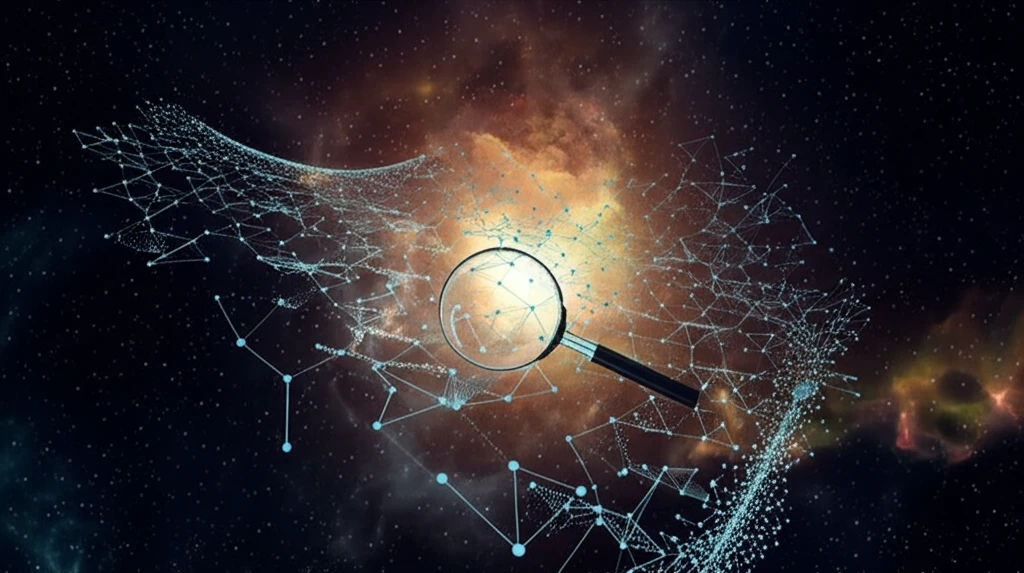
Dyadic data focuses on pairwise relationships or interactions. Examples include trade volumes between countries, migration flows between regions, social networks within organizations, and even disease transmission between individuals. The key characteristic is that each data point represents a connection between two entities.
- Network Formation Processes: The underlying mechanisms that create or inhibit relationships. For example, geographical distance, cultural similarities, or existing agreements can influence trade relationships.
- Data Collection Limitations: Practical constraints that prevent the observation of all possible pairs. This could be due to cost, logistical challenges, or privacy concerns.
- Strategic Decisions: Intentional choices made by actors that create or break relationships. For instance, companies might strategically choose to form partnerships with certain organizations based on specific objectives.
- Inaccurate Estimates: Biased coefficients in regression models, leading to incorrect inferences about the factors that drive dyadic relationships.
- Flawed Predictions: Poor predictive performance when extrapolating models to unseen data or new contexts.
- Misguided Decisions: Incorrect conclusions that inform ineffective policies or business strategies.
Embrace Robust Analysis for Reliable Insights
Dyadic data offers a powerful lens for understanding relationships and interactions in various domains. By acknowledging and addressing the challenges of sample selection bias, you can unlock the full potential of this data and gain reliable, actionable insights. Embrace the techniques outlined in this guide to improve the accuracy of your models, enhance the robustness of your findings, and drive data-informed decisions with confidence. Whether you’re mapping global trade, understanding social networks, or analyzing complex systems, a rigorous approach to dyadic data analysis will set you on the path to success.