Decoding Digital Journeys: How Websites Learn Your Preferences with AI
"Unlocking the secrets of user navigation behavior with colored Petri nets to deliver personalized web experiences."
In today's vast digital landscape, finding the information you need can feel like searching for a needle in a haystack. As the web continues to expand, search engines and websites are constantly evolving to meet the challenge of delivering relevant content to users efficiently. One of the most promising approaches involves understanding and modeling user navigation behavior.
Traditionally, search engines have relied on two primary methods: analyzing user feedback and tracking user interests through statistical information. However, these methods often require explicit user input, which can be time-consuming and deter many users. A more effective approach is to passively extract user interests from their browsing behavior and create personalized web experiences based on those insights.
This article delves into the fascinating world of web personalization and explores how advanced modeling techniques, such as colored Petri nets, are being used to understand user navigation behavior and deliver tailored content. We'll examine the benefits of this approach, its applications, and the future of personalized web experiences.
How Do Websites Learn Your Preferences?
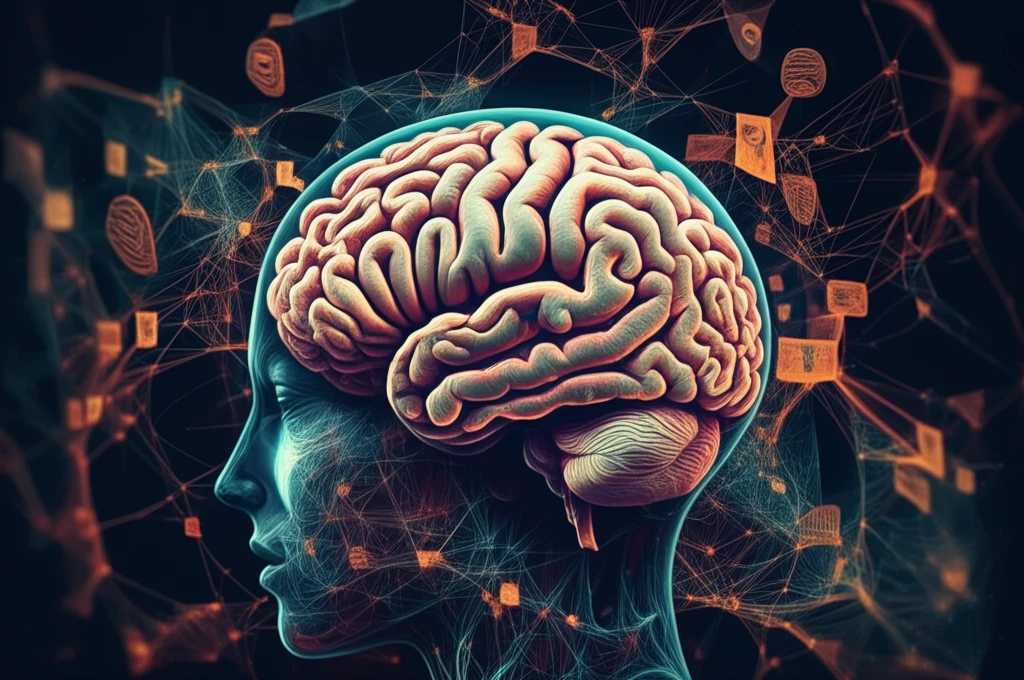
Web personalization aims to provide users with the content they need in an optimal way. By analyzing user behavior and data from previous interactions, websites can identify patterns and recommend services, products, and information tailored to individual needs. This approach relies on sophisticated techniques to model user navigation and predict their interests.
- User Feedback Systems: Analyze explicit feedback provided by users.
- Interest Tracking: Record user interests and statistical information.
- Behavioral Analysis: Extract user interests from browsing patterns.
The Future of Personalized Web Experiences
As AI continues to advance, web personalization is poised to become even more sophisticated. By combining advanced modeling techniques with machine learning algorithms, websites will be able to create truly personalized experiences that anticipate user needs and deliver the right content at the right time. This will not only improve user satisfaction but also drive engagement and conversions.