Decoding Detection: How Union of Subspaces Revolutionizes Signal Processing
"Unlock the secrets of advanced signal analysis: Discover how geometric insights and innovative testing are changing detection theory for diverse applications."
Imagine trying to find a specific radio station amidst a cacophony of signals, or identifying a face in a crowd under varying lighting conditions. Traditional signal processing often falls short in these complex scenarios. Classical detection theory, rooted in the subspace model, assumes signals neatly fit into low-dimensional spaces, but real-world data rarely behaves so predictably.
Enter the Union of Subspaces (UoS) model, a sophisticated approach that's gaining traction for its ability to better represent the intricate nature of real-world signals. In essence, the UoS model acknowledges that data is often generated by processes that operate in different modes, each corresponding to a unique subspace. This framework allows for a more flexible and accurate representation of complex signals, opening doors to enhanced detection capabilities.
This article dives into the core concepts of detection theory for the Union of Subspaces model. We’ll breakdown how to determine whether a signal aligns with a known generation mechanism and how to identify the active subspace or mode that produced it. This method provides a comprehensive understanding of this emerging field and its potential applications in various domains.
The Power of GLRTs in UoS Detection
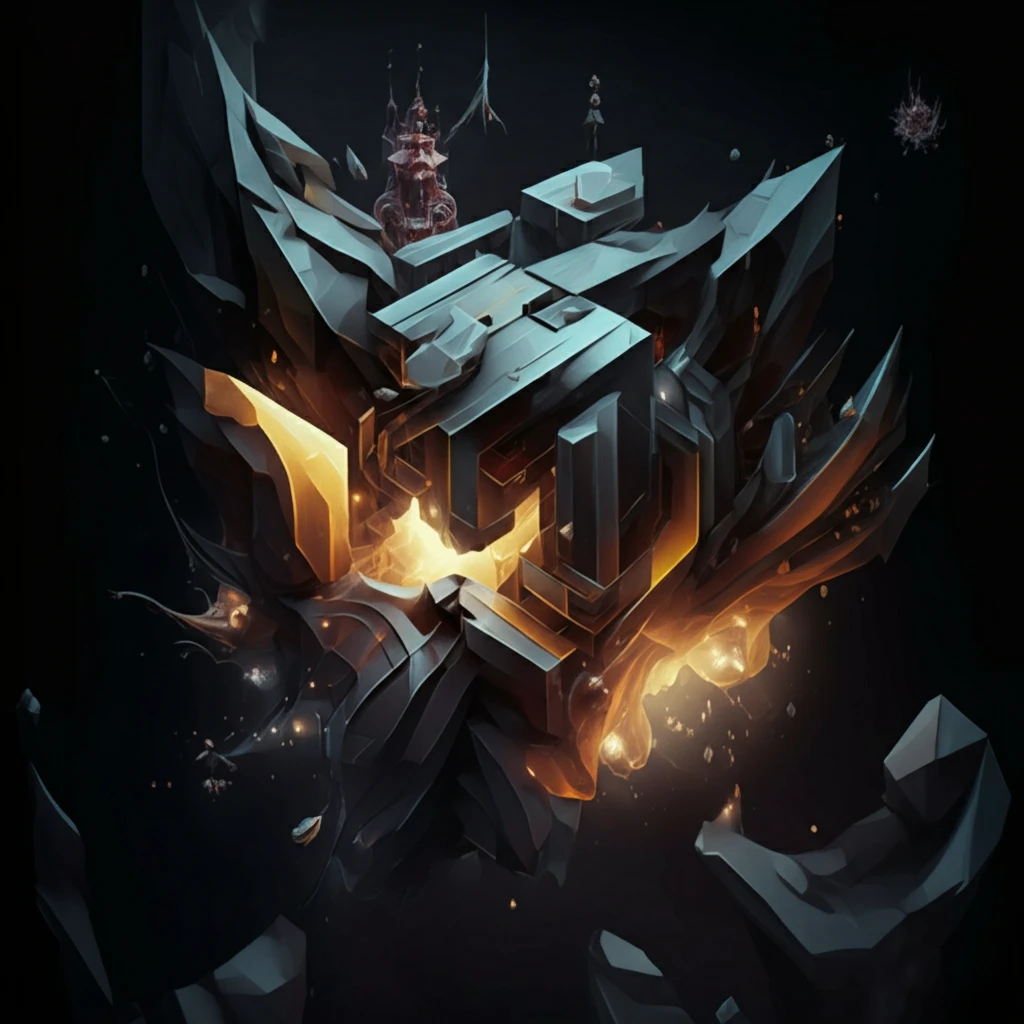
At the heart of UoS detection lies the Generalized Likelihood Ratio Test, or GLRT. This statistical test helps determine the most likely hypothesis given observed data. In the context of UoS, GLRTs are used to detect signals conforming to the UoS model and pinpoint the corresponding "active" subspace, this becomes valuable when determining signal origins. One of the key contributions is the establishment of performance bounds for these GLRTs, framed by the geometry of subspaces and different assumptions about observation noise.
- Enhanced Accuracy: GLRTs offer a more accurate way to identify signals in complex data.
- Geometric Insight: The GLRT method is able to interpret information using geometrical shapes.
- Noise Handling: GLRT’s have abilities to handle different forms of noise.
- Versatile Applications: Applicable for anything from spectral analysis, to wireless communications, to identifying an employee.
Looking Ahead
The Union of Subspaces model and the GLRT offer a powerful new lens through which to view signal detection. By embracing the complexity of real-world data and leveraging geometrical insights, these methods pave the way for more accurate and robust detection systems. As research continues, we can expect to see even wider applications of UoS in diverse fields, transforming how we process and interpret signals in an increasingly complex world.