Decoding Data: How Trimmed Means Help Us Make Sense of Complex Datasets
"Explore how halfspace depth trimmed means offer a robust approach to data analysis, mitigating the impact of outliers and revealing underlying patterns."
In our increasingly data-driven world, making sense of complex datasets is crucial. Traditional measures like the mean can be easily skewed by outliers, leading to inaccurate conclusions. This is where trimmed means come in as a more robust alternative. Trimmed means offer a way to mitigate the impact of extreme values, providing a clearer picture of the underlying data trends.
Imagine you're analyzing customer satisfaction scores, and a few extremely negative reviews disproportionately drag down the average. A trimmed mean would exclude these extreme scores, giving you a better sense of the typical customer experience. This concept extends beyond simple averages, with methods like halfspace depth trimmed means offering powerful tools for analyzing multivariate data.
This article explores the concept of trimmed means, focusing on the innovative approach of general halfspace depth trimmed means. We'll delve into how these methods work, their theoretical underpinnings, and why they're becoming increasingly important in various fields that rely on robust statistical analysis.
What are Halfspace Depth Trimmed Means and Why Do They Matter?
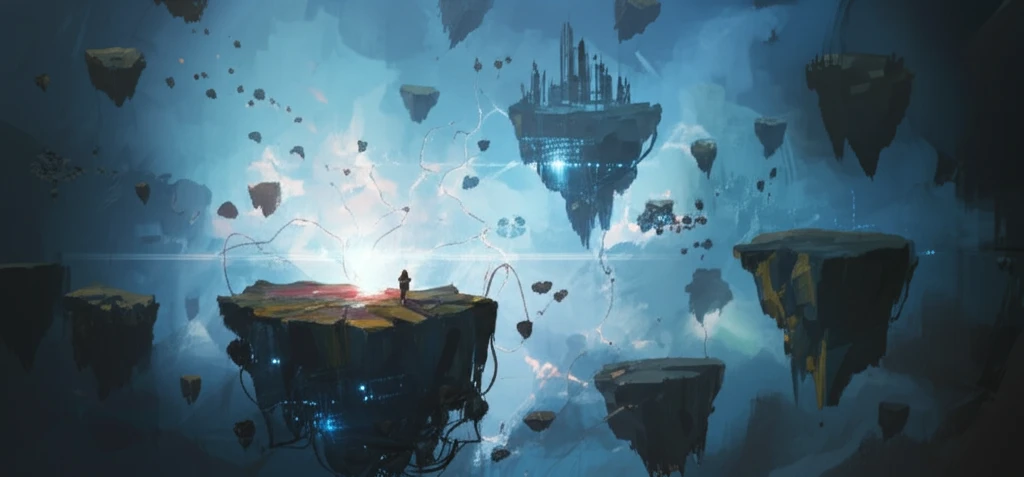
The standard trimmed mean, commonly used in univariate data, involves removing a certain percentage of the lowest and highest values before calculating the average. Halfspace depth trimmed means extend this concept to multivariate data, which involves multiple variables. This extension is based on the idea of 'depth,' which measures how 'central' a point is within a dataset.
- Robustness: Trimmed means are less sensitive to outliers, providing a more stable estimate of central tendency.
- Efficiency: By excluding extreme values, trimmed means can sometimes be more efficient than the standard mean, especially in datasets with heavy tails.
- Flexibility: The trimming percentage can be adjusted to balance robustness and efficiency, depending on the specific characteristics of the data.
- Multivariate Analysis: Halfspace depth extends the concept to higher dimensions.
The Future of Data Analysis: Embracing Robust Methods
As datasets grow larger and more complex, the need for robust statistical methods will only increase. Halfspace depth trimmed means offer a powerful tool for navigating the challenges of outlier-prone data, enabling more accurate and reliable insights. By embracing these techniques, we can unlock the full potential of our data and make better-informed decisions.