Decoding Data: How to Make Sense of Massive Datasets and Variance Components
"Unlock the Secrets Hidden Within Large Datasets: A Practical Guide to Variance Components and Efficient Analysis Techniques"
In today's digital age, we're constantly bombarded with information. From tracking customer behavior to analyzing website traffic, massive datasets are becoming increasingly common. However, making sense of this data can feel like trying to find a needle in a haystack. The sheer volume and complexity can be overwhelming, especially when you're trying to identify key trends and insights.
One of the biggest challenges is figuring out how much variation exists within the data and where that variation comes from. This is where the concept of "variance components" comes into play. Variance components help us understand how different factors contribute to the overall variability in a dataset, allowing us to pinpoint the most influential drivers and make more accurate predictions.
Traditional statistical methods often struggle to handle these large, complex datasets efficiently. Many techniques take a superlinear time to process the data in proportion to its size. That’s like if it takes 2 days to process 2000 rows of data, it would take a whooping 6 days to process 3000 rows. This is why we need new approaches that can scale effectively and provide insights without breaking the bank or wasting precious time. Let’s dive into some efficient techniques for calculating variance components and unlocking the hidden potential within your data.
What Are Variance Components and Why Should You Care?
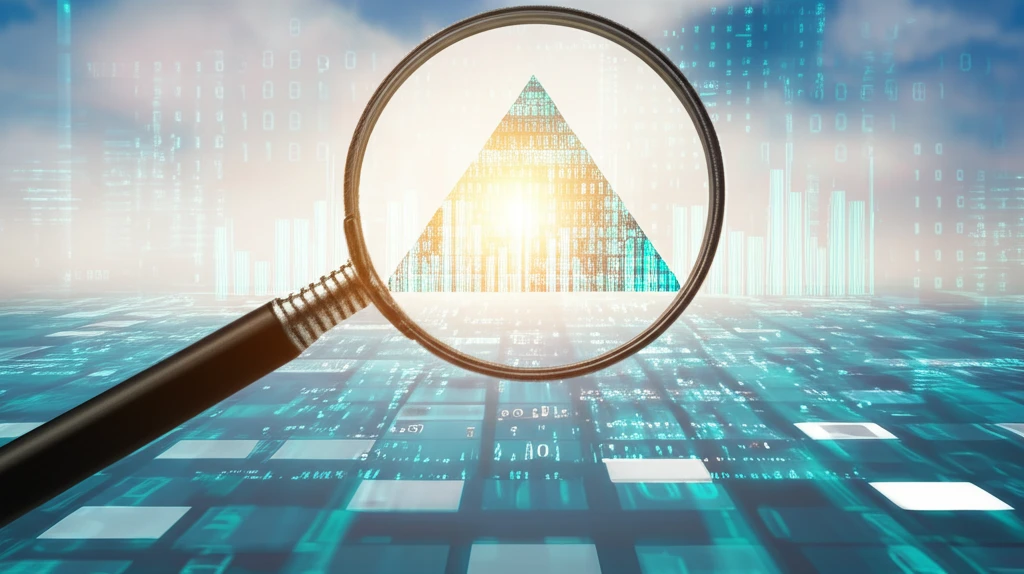
Variance components are statistical measures that tell us how much of the total variance in a dataset is due to different sources of variation. Think of it like a pie chart where each slice represents the proportion of variance contributed by a specific factor. In simpler terms, variance components help us break down the noise and identify the key drivers influencing our data.
- How much of the variation in scores is due to individual customer differences (some people are just naturally more critical than others)?
- How much is due to product-specific features (some features might be universally loved while others are polarizing)?
- How much is due to random error or unexplained factors?
The Future of Data Analysis: Scalable and Efficient Methods
The ability to efficiently analyze large datasets and understand variance components is becoming increasingly important in today's data-driven world. While traditional methods may struggle to keep up, new approaches are emerging that can provide insights without breaking the bank or wasting precious time. By embracing these techniques, you can unlock the hidden potential within your data and make more informed decisions.