Decoding Data: How to Handle Uncertainty and Set Meaningful Limits
"Navigate the complexities of data analysis with confidence. Learn practical Bayesian methods to manage systematic errors and set informed limits for clearer insights."
Data analysis can often feel like navigating a maze of statistical recipes, each seeming disconnected and lacking a clear rationale. Many prescriptions appear arbitrary because they lack a unifying principle, making the underlying assumptions and approximations unclear.
While guidelines for 'best practices' are helpful, they often fall short without a solid educational foundation in the fundamental principles of data analysis. Scientists and engineers are increasingly turning to the Bayesian approach to probability theory, championed by mathematical physicists like Laplace, Jeffreys, and Jaynes, as a more suitable framework.
This method provides a coherent way to deal with uncertainty and set limits on inferred parameters. We'll explore this viewpoint, provide an instructive example of dealing with systematic uncertainties, and offer a novel suggestion for setting limits.
Bayesian Probability: A Clearer Lens
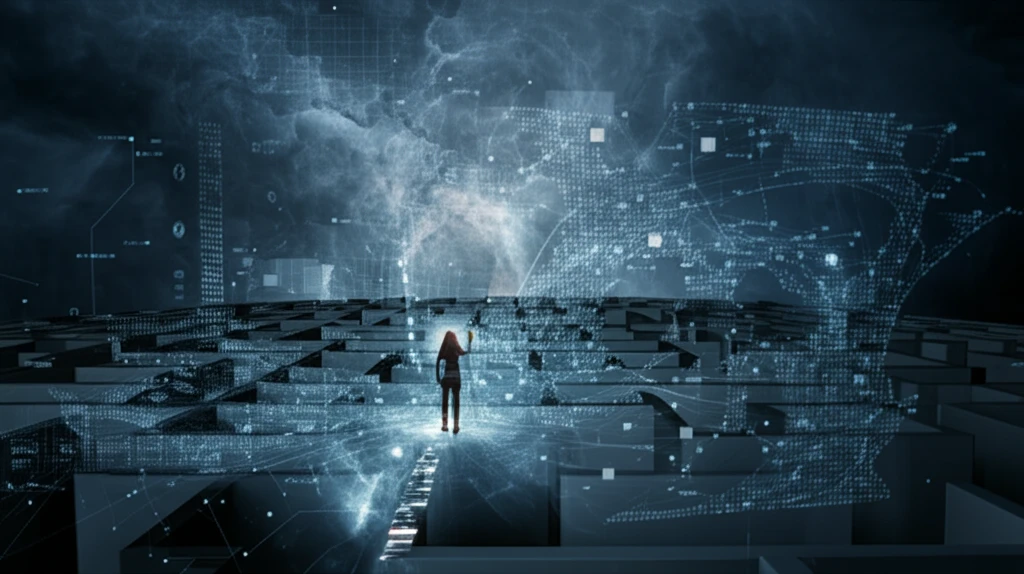
The Bayesian approach to probability theory, dating back over three hundred years, provides a robust tool for reasoning in uncertain situations. Unlike classical statistics, which primarily focuses on frequencies, Bayesian probability represents a degree of belief or a state of knowledge given available information.
- Conditional Probability: Probabilities are always assessed in the context of specific information.
- Degree of Belief: Probabilities represent how confident we are in an event.
- Dynamic Updates: Probabilities can be updated as new information becomes available.
Embracing Uncertainty with Clarity
Adopting a Bayesian approach to data analysis provides a powerful framework for making informed decisions in the face of uncertainty. By understanding the principles outlined here, you can move beyond black-box methodologies and gain deeper insights from your data. Remember, every analysis is conditional on assumptions, so understanding and clearly stating these conditions is essential for sound and reliable conclusions.