Decoding Data: How New Matrix Methods Are Changing What We Know
"Discover how orthogonal nonnegative matrix tri-factorization is revolutionizing data analysis for more accurate insights."
In an era defined by unprecedented data volume, the tools we use to analyze this information are more crucial than ever. Traditional methods often fall short when dealing with complex datasets, leading to inaccuracies and skewed interpretations. Enter orthogonal nonnegative matrix tri-factorization (ONMTF), an advanced technique that's reshaping how we approach data analysis.
ONMTF serves as a powerful biclustering method, adept at dissecting nonnegative data matrices. Its applications span various fields, from document-term clustering to collaborative filtering. But ONMTF's true potential lies in its ability to overcome the limitations of previous models that assume a normal distribution—an assumption often unsuitable for real-world data.
Recent advancements in ONMTF have introduced innovative methods that employ alternative error distributions, such as Poisson and compound Poisson. These approaches, coupled with k-means-based algorithms, enhance the accuracy and robustness of data clustering and factor matrix estimation. This article explores the intricacies of ONMTF and its transformative impact on data analysis.
The Power of Orthogonal Nonnegative Matrix Tri-Factorization
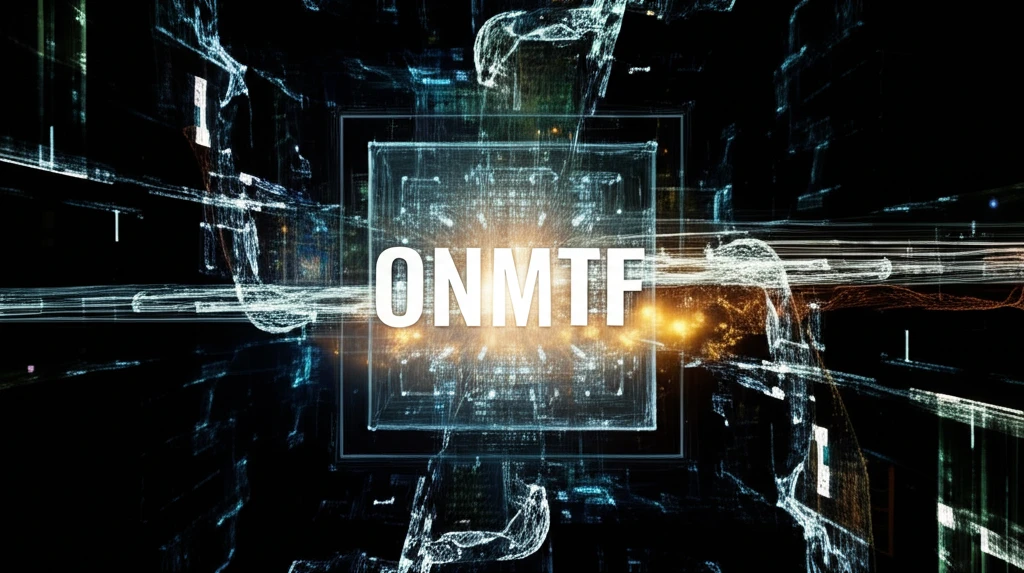
ONMTF stands out because it addresses a critical flaw in conventional data analysis: the assumption of normally distributed errors. This assumption, while convenient, often fails to capture the true nature of nonnegative data, leading to suboptimal results. By incorporating different error distributions, ONMTF provides a more flexible and accurate framework for data interpretation.
- Enhanced accuracy in data clustering.
- Improved robustness against outliers.
- Greater flexibility in handling different types of data.
- More meaningful interpretations of complex datasets.
The Future of Data Interpretation
As data continues to grow in both volume and complexity, ONMTF offers a promising path forward for more accurate and meaningful data interpretation. By moving beyond the limitations of traditional methods and embracing new error distributions and algorithmic approaches, ONMTF is set to become an indispensable tool for data scientists and analysts across various domains. Further research and development in this area promise even greater insights and applications in the years to come.