Decoding Data: How Hamiltonian Monte Carlo Is Revolutionizing Economic Research
"Unlock the power of advanced statistical methods to transform high-dimensional categorical data into actionable insights."
In today's data-rich environment, the ability to extract meaningful information from complex data is crucial for progress in various fields. Economics is no exception. As the volume of digitally recorded unstructured data continues to surge, economic researchers are increasingly incorporating diverse data types, such as text, surveys, images, and audio recordings, into their analyses. The challenge, however, lies in effectively handling the high dimensionality and categorical nature of such data.
One common approach is to use statistical models that project high-dimensional data onto a lower-dimensional space, capturing the essential patterns. For instance, in natural language processing, Latent Dirichlet Allocation (LDA) is a popular method for uncovering underlying topics within large text corpora. Yet, these methods often serve as a preliminary step, with researchers subsequently using the transformed data in regression models. This two-step approach introduces methodological issues, potentially leading to inaccurate inferences and inefficient analyses.
Recent research published on arXiv.org explores a more integrated approach using Hamiltonian Monte Carlo (HMC). This method jointly specifies and estimates latent variable models and regression models within a single data-generating process. The study highlights how HMC, combined with parallelized automatic differentiation, can efficiently analyze high-dimensional categorical data, offering a more robust and insightful alternative to traditional methods.
Why Traditional Methods Fall Short: Understanding the Limitations
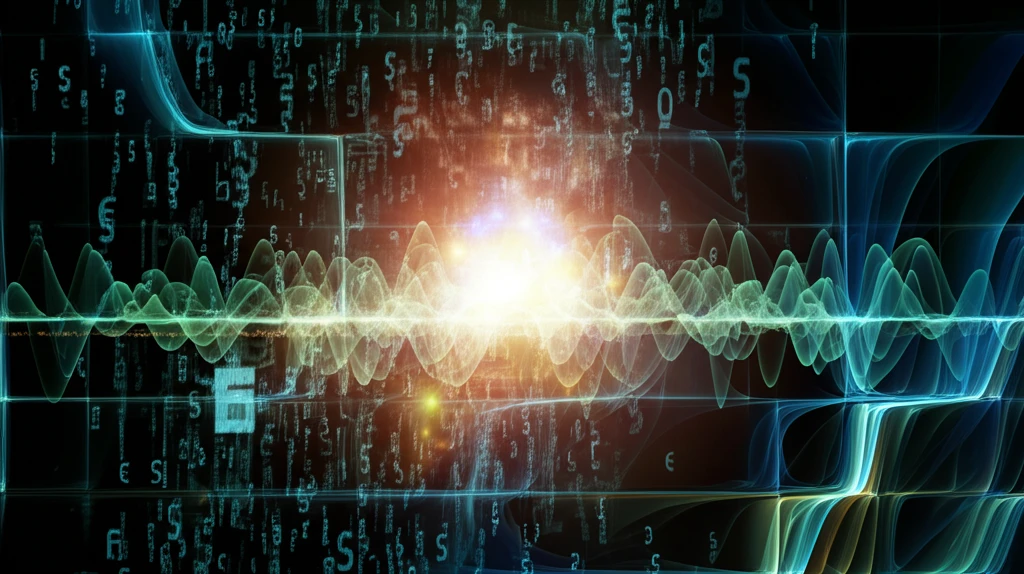
The conventional two-step approach to analyzing unstructured data involves first transforming the data into a manageable numeric form and then using it in a regression model. However, this method has several drawbacks. Uncertainty from the initial transformation step is often ignored, leading to invalid inferences. Weighting observations equally can be inefficient when estimates vary in precision, and the regression model may impose unrealistic dependencies between latent representations and covariates.
- Ignoring Uncertainty: The initial step of transforming unstructured data introduces uncertainty that is often disregarded in subsequent regression analyses.
- Inefficient Weighting: Traditional methods may weigh all observations equally, even when the precision of individual estimates varies significantly.
- Oversimplification: Regression models can impose unrealistic dependencies between latent representations and covariates, potentially distorting the true relationships.
The Future of Data Analysis: Embracing Integrated Methodologies
As the volume and complexity of data continue to grow, the need for advanced analytical techniques like Hamiltonian Monte Carlo will become increasingly critical. By moving beyond traditional two-step approaches and embracing integrated methodologies, researchers can unlock deeper insights, make more accurate predictions, and drive innovation across various fields. The ability to efficiently analyze high-dimensional categorical data is no longer a luxury but a necessity for staying ahead in today's data-driven world. The integration of unstructured data with HMC offers a promising pathway for the developments of new discoveries.