Decoding Data Chaos: How 'Compounding' Can Predict the Unpredictable
"Unlock hidden patterns in noisy data using the compounding approach, a powerful tool for understanding everything from turbulent air to fluctuating stock prices."
We're constantly bombarded with data, but much of it seems random. Whether it’s the erratic gusts of wind from a fan or the dizzying ups and downs of the stock market, these phenomena often appear too chaotic to understand. Traditional statistical methods often fall short when dealing with such 'non-stationary' systems, where the underlying parameters change over time.
Enter the 'compounding approach,' also known as 'superstatistics.' This technique provides a framework for analyzing these complex systems by recognizing that what appears random on the surface might be governed by a hidden order. It's like looking at a turbulent river and realizing that, even though the water is swirling, there are underlying currents and eddies that shape its flow.
This approach doesn't magically make the unpredictable predictable, but helps in creating models that are significantly more accurate by considering that the underlying parameters of these systems are not fixed.
What is Compounding, and Why Does It Matter?
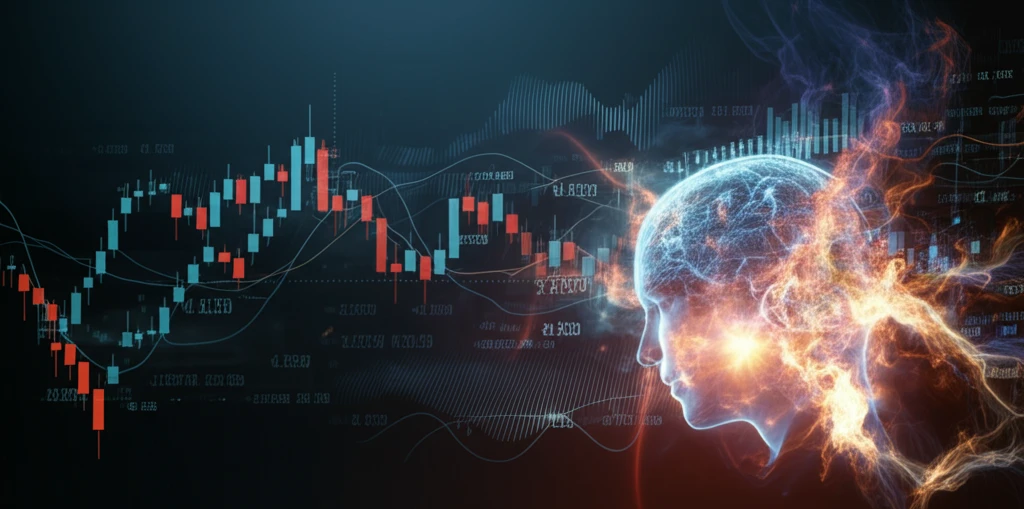
At its heart, compounding involves recognizing that a system's overall behavior is a mixture of different statistical states. Imagine that turbulent air flow again. On a very short timescale, the air's velocity might seem to follow a normal (Gaussian) distribution. However, over longer periods, the variance (a measure of how spread out the data is) changes. Compounding acknowledges this by averaging the local Gaussian distributions over the distribution of the variance.
- Handles Non-Stationarity: It directly addresses systems where statistical properties change over time.
- Reveals Hidden Structure: It uncovers underlying patterns masked by apparent randomness.
- Improves Modeling: It leads to more accurate predictions than traditional methods that assume fixed parameters.
- Applies Broadly: It can be used across diverse fields, from physics to finance.
Navigating Data's Complexity
The compounding approach offers a versatile toolkit for grappling with the inherent unpredictability of complex systems. By explicitly acknowledging the time-varying nature of statistical parameters and adopting an empirical approach to characterizing variance distributions, this methodology equips analysts and researchers with enhanced capabilities to model, forecast, and manage risk in an increasingly turbulent world. As our capacity to gather data continues to grow, methodologies such as compounding will play a pivotal role in discerning valuable insights and patterns from the noise.