Decoding Cooperation: How Reinforcement Learning Shapes Our Social Interactions
"Uncover the surprising ways that reinforcement learning influences cooperation and decision-making in complex social environments."
The puzzle of cooperation has captivated researchers across diverse fields, from biology to economics. Understanding how cooperation emerges and persists is crucial for addressing many of society's most pressing challenges. Two primary factors influencing cooperative behavior are the structure of interactions (who interacts with whom) and the mode of cognition (the degree of deliberation versus intuition).
Traditionally, studies have focused on behavioral rules like 'best reply' or 'imitation.' However, the rise of reinforcement learning (RL), a powerful tool from computer science, offers a fresh perspective. RL allows agents to learn optimal strategies through trial and error, adapting to their environment based on rewards and penalties. But how does RL impact cooperation in complex social settings?
A recent study delves into this question by examining the coevolution of cognition and cooperation in structured populations. By integrating reinforcement learning into a classic game theory model—the Prisoner's Dilemma—the researchers uncover surprising insights into how learning, network structure, and deliberation shape cooperative outcomes.
Reinforcement Learning and the Prisoner's Dilemma: A New Perspective
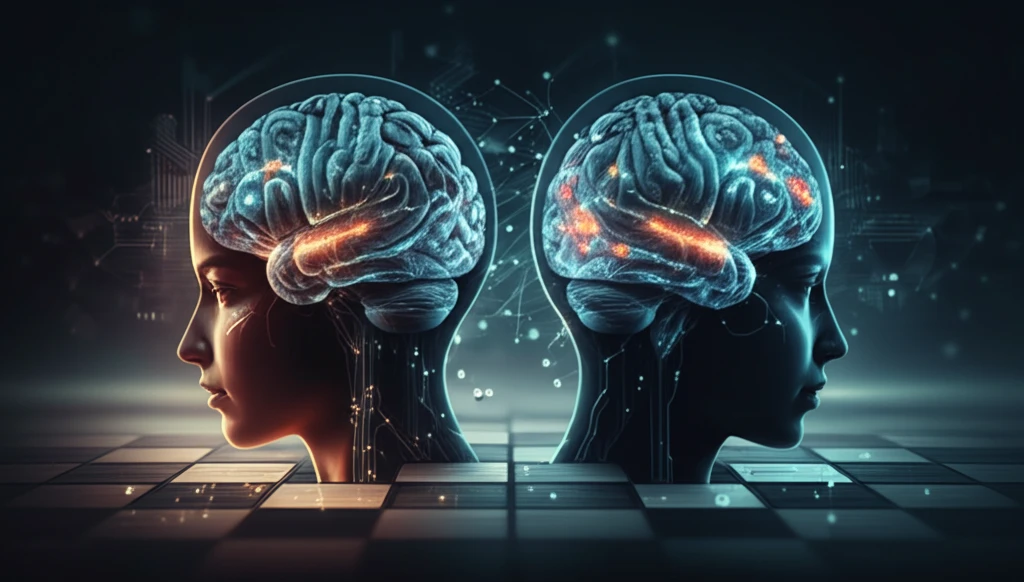
The study builds upon existing models of cooperation, such as those by Mosleh and Rand, by incorporating a k-regular lattice structure, where each agent interacts with a fixed number of neighbors. Unlike models with pre-defined strategies, agents in this model use reinforcement learning to adapt their behavior based on past experiences. Agents learn whether to play a one-shot or a repeated game by incurring a cost of deliberation.
- Confirmation of previous results: Consistent with earlier research, the study confirms the existence of a threshold value for the probability of repeated interaction. Below this threshold, intuitive defection dominates, while above it, dual-process cooperation (cooperating unless deliberation suggests defection) prevails.
- The role of node degree: Contrary to some previous findings, the study reveals that smaller node degrees (fewer connections) reduce the evolutionary success of dual-process cooperators, making intuitive defection more likely.
- Increased deliberation: Reinforcement learning leads to a higher frequency of deliberation, suggesting that even with a cognitively cheap behavioral rule, agents rely more on careful consideration.
Implications and Future Directions
This research underscores the importance of considering reinforcement learning when studying cooperation. While the threshold for switching from intuitive defection to dual-process cooperation remains consistent, the influence of network connections is moderated by the behavioral rule. Future research could explore how dynamically evolving networks and co-evolving cognition and cooperation further shape social interactions, offering a richer understanding of how cooperation emerges and thrives in complex social systems.