Decoding Brain Scans: Can AI Texture Analysis Improve Glioma Diagnosis?
"Explore how AI-driven texture analysis enhances MRI interpretation for glioma classification, potentially revolutionizing brain tumor diagnostics for better patient outcomes."
Gliomas, tumors originating in the brain's glial cells, present a significant diagnostic challenge due to their varied nature. Accurate classification of these tumors is crucial as it directly impacts treatment strategies and patient prognosis. Traditionally, this classification relies on histopathological examination and genetic analysis, coupled with the interpretation of Magnetic Resonance Imaging (MRI) scans.
However, MRI interpretation can be subjective and may miss subtle yet critical details. This is where texture analysis steps in—a sophisticated method that uses algorithms to quantify the textural patterns within an image that might be imperceptible to the human eye. Recent advancements have seen the integration of artificial intelligence (AI) to enhance this analysis, promising a more objective and accurate diagnostic process.
This article delves into how AI-enhanced texture analysis is transforming the diagnostic landscape for gliomas. By examining a recent study that explores the application of AI in classifying low-grade gliomas (LGGs) using MRI data, we shed light on the potential benefits of this innovative approach.
How Does Texture Analysis Work in Glioma Diagnosis?
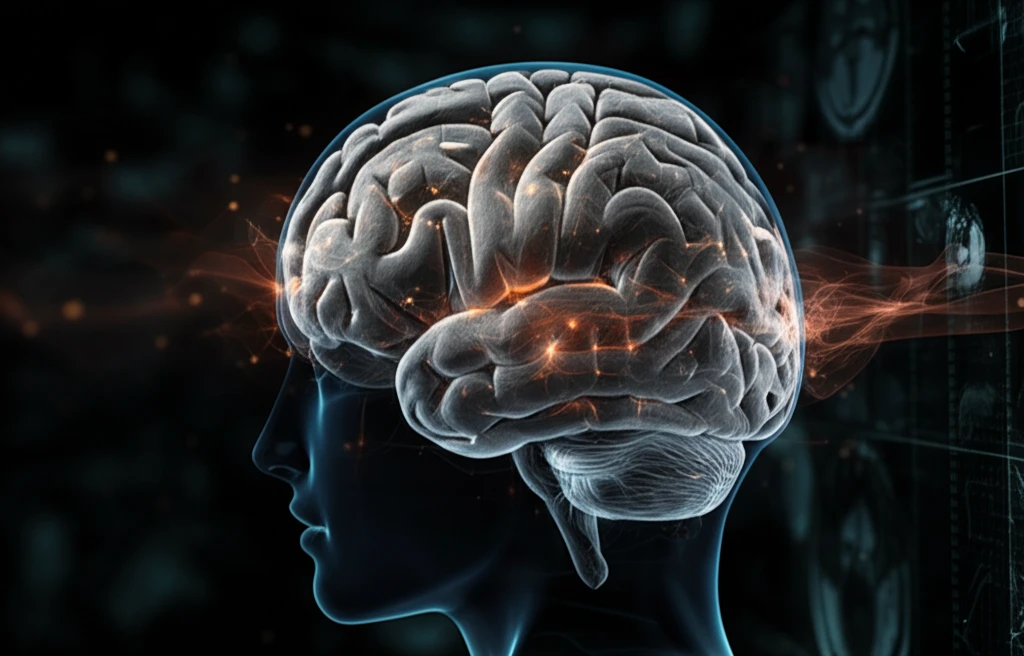
Texture analysis works by examining the spatial relationships of pixel intensities in an MRI image. In the context of glioma diagnosis, it helps to identify and quantify characteristics such as homogeneity, contrast, and structural arrangements within the tumor. These features can then be correlated with different grades and types of gliomas, providing valuable diagnostic insights.
- Data Collection: MRI scans from 41 patients with LGGs were used, including both astrocytomas and oligodendrogliomas.
- Image Analysis: Texture analysis was performed on various MRI sequences, including T2-weighted FLAIR, ADC, T1-weighted, and T1-weighted with gadolinium.
- Feature Extraction: The analysis extracted 279 texture features from each MRI image, encompassing statistical measures like histogram features, gradient features, and run-length matrices.
- AI Integration: Machine learning algorithms were employed to identify the most discriminant features, enhancing the accuracy of tumor classification.
The Future of Glioma Diagnostics with AI
The integration of AI into texture analysis represents a significant leap forward in the diagnosis and classification of gliomas. By providing a more objective, accurate, and efficient method for analyzing MRI data, this technology has the potential to improve patient outcomes through better-informed treatment decisions. As AI continues to evolve, its role in medical imaging and diagnostics will likely expand, paving the way for more personalized and effective healthcare strategies.