Decoding Assembly Lines: How Smart Tech Can Streamline Your Production
"Unlock efficiency with cutting-edge machine learning. See how optimized assembly lines boost productivity and reduce costs for a more agile operation."
In today's fast-paced manufacturing landscape, the assembly line remains a critical component for producing goods efficiently. However, traditional assembly lines can often be rigid, slow to adapt to changes, and prone to bottlenecks. This is where the power of machine learning (ML) comes into play, offering innovative solutions to optimize and revolutionize the entire assembly process.
Imagine an assembly line that can dynamically adjust to fluctuations in demand, predict potential equipment failures before they occur, and automatically reconfigure itself to handle new product variations. This isn't a futuristic fantasy; it's the reality that machine learning is making possible right now. By leveraging data-driven insights, manufacturers can create assembly lines that are not only faster and more efficient but also incredibly agile and resilient.
This article delves into the fascinating world of machine learning applications in assembly line optimization. We'll explore the challenges of traditional assembly line balancing, how ML algorithms are addressing these issues, and the tangible benefits that manufacturers can achieve by embracing these technologies. Get ready to discover how smart tech is transforming the way products are made, one assembly line at a time.
The Challenge of Balancing Act
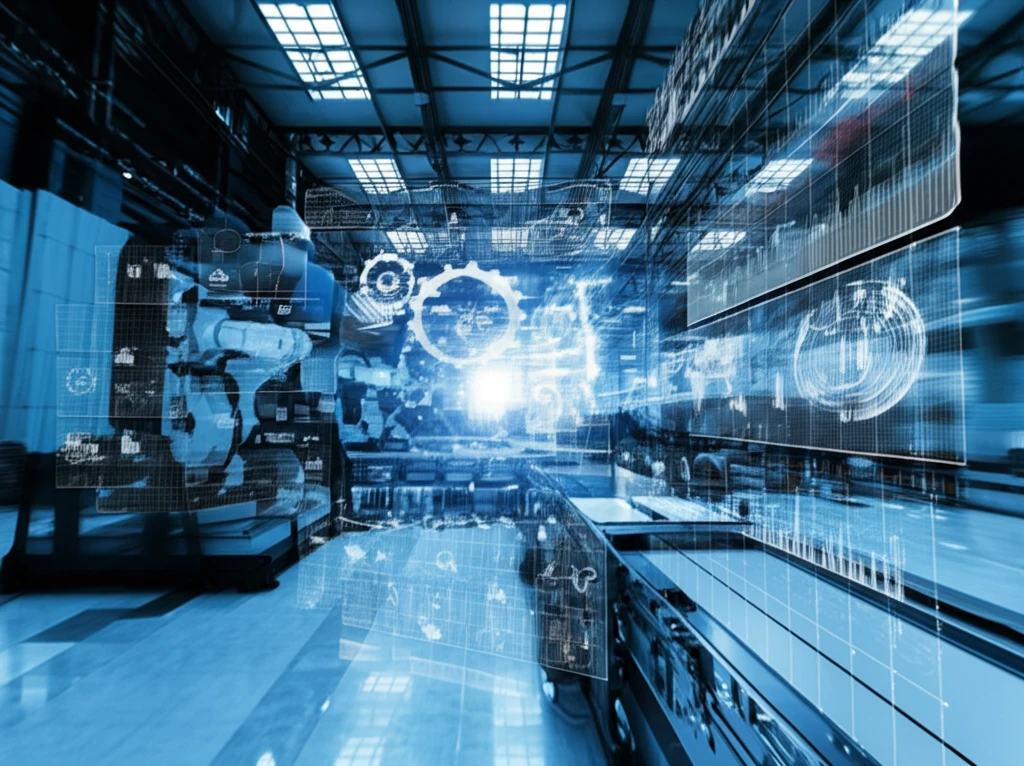
The traditional assembly line balancing problem (ALBP) is a complex puzzle. It involves assigning tasks to workstations in a way that minimizes idle time, maximizes efficiency, and adheres to various constraints, such as task precedence (some tasks must be completed before others) and cycle time (the maximum time allowed for each station to complete its tasks). The goal is to distribute the workload evenly across all stations, ensuring a smooth and continuous flow of production.
- Adaptability: ML algorithms can learn from real-time data and dynamically adjust assembly line configurations to optimize performance under changing conditions.
- Predictive Maintenance: ML models can analyze sensor data from equipment to predict potential failures, allowing for proactive maintenance and minimizing downtime.
- Worker Skill Optimization: ML can identify optimal task assignments based on individual worker skills and experience, improving overall productivity.
- Improved Throughput: ML algorithms can optimize material flow and task sequencing to minimize bottlenecks and increase throughput.
The Future of Assembly Lines
The integration of machine learning into assembly line design and operation is still in its early stages, but the potential benefits are undeniable. As ML algorithms become more sophisticated and data availability increases, we can expect to see even more innovative applications emerge, further transforming the manufacturing landscape. Embracing these technologies will be crucial for manufacturers seeking to stay competitive, improve efficiency, and adapt to the ever-changing demands of the modern marketplace. The journey towards smarter, more agile assembly lines has only just begun.