Decoding Algorithmic Bias: Can We Fix the Code?
"Uncover the surprising ways algorithms perpetuate discrimination and explore innovative interventions for a fairer future."
In our increasingly digital world, algorithms are the invisible hands shaping countless decisions, from loan applications and hiring processes to college admissions and even criminal justice. But what happens when these supposedly objective systems perpetuate existing societal biases, leading to unfair or discriminatory outcomes? This is the challenge of algorithmic bias, a problem that's gaining increasing attention as AI and machine learning become more deeply integrated into our lives.
Algorithmic bias arises when algorithms, trained on biased data or designed with flawed assumptions, systematically favor certain groups over others. This can have far-reaching consequences, reinforcing inequalities and limiting opportunities for marginalized communities. While the problem is complex, researchers are exploring innovative interventions to mitigate algorithmic bias and promote fairer outcomes.
This article delves into the issue of machine-assisted statistical discrimination, drawing on insights from a groundbreaking study. We'll explore how algorithms learn and perpetuate bias, and what steps can be taken to ensure that these powerful tools are used to create a more equitable future.
The Hidden Ways Algorithms Learn and Reinforce Bias
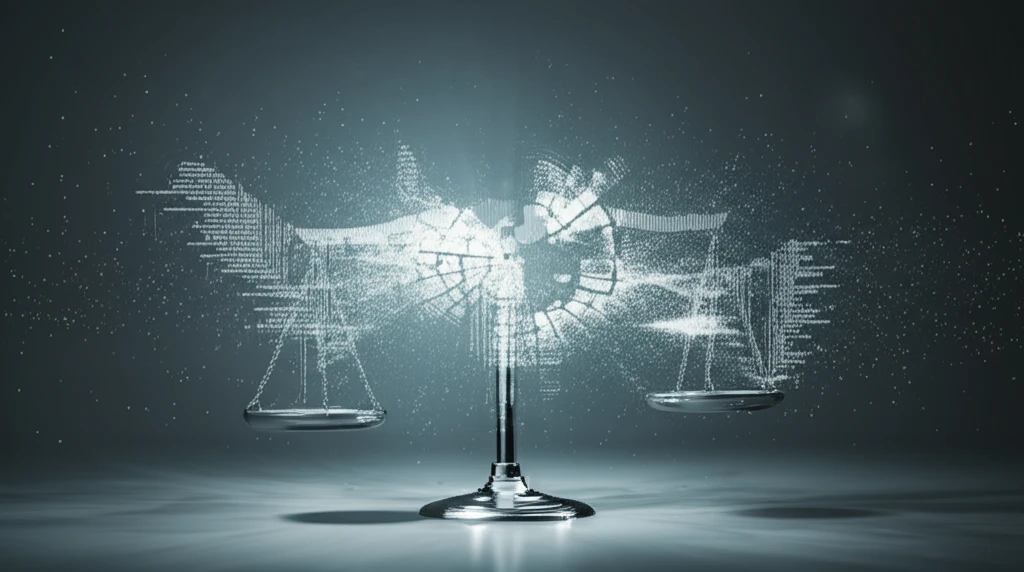
At the heart of algorithmic bias lies the data used to train these systems. Machine learning algorithms learn by identifying patterns in data, and if that data reflects existing societal biases, the algorithm will inevitably replicate those biases in its decision-making. For example, if a hiring algorithm is trained on historical data where men were predominantly hired for certain roles, it may learn to favor male applicants, even if they are less qualified than their female counterparts.
- Data Bias: Historical data reflecting societal inequalities.
- Flawed Assumptions: Design choices that inadvertently discriminate.
- Feedback Loops: Biased outcomes reinforcing existing prejudices.
Toward a Fairer Algorithmic Future
Combating algorithmic bias requires a multi-faceted approach that addresses both the data and the design of these systems. It demands careful attention to the data used for training, rigorous testing for discriminatory outcomes, and ongoing monitoring to ensure fairness over time. The interventions discussed in this article offer promising pathways towards mitigating algorithmic bias and creating a future where AI benefits everyone, not just a privileged few. As AI continues to evolve, it's crucial that we prioritize fairness and equity, ensuring that these powerful tools are used to build a more just and inclusive society.