Cracking the Code: How Personalized Medicine and AI are Revolutionizing Diabetes Treatment
"Discover how matched learning and electronic health records (EHRs) are paving the way for individualized treatment strategies for Type 2 Diabetes, offering hope for better outcomes."
For years, medical treatments have often followed a one-size-fits-all approach, guided by the average results seen in clinical trials. However, everyone knows that each person's body is different, and what works for one patient may not work for another. This is especially true for complex conditions like type 2 diabetes (T2D), where managing blood sugar and preventing complications requires personalized strategies.
The rise of electronic health records (EHRs) offers a sea change, providing vast amounts of real-world patient data. Experts can now use this information to develop individualized treatment rules (ITRs) that consider a patient's unique characteristics and history. One exciting approach is "matched learning," a type of machine learning that leverages EHR data to optimize treatment strategies.
Imagine a future where doctors use AI to analyze your health records and pinpoint the precise treatment plan that suits your needs. This future is closer than you think, and it's being driven by innovations in machine learning and the increasing availability of detailed patient data.
What is Matched Learning and Why is It a Game Changer for Diabetes?
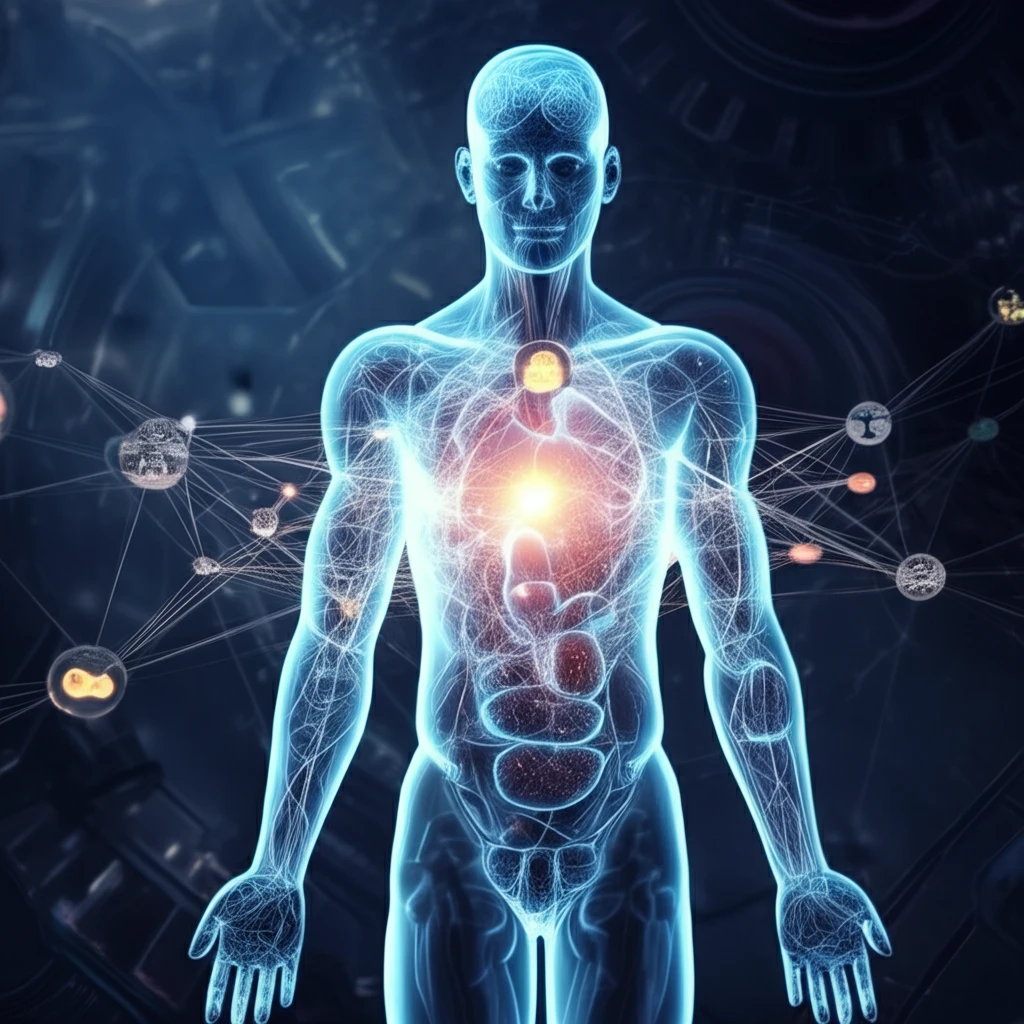
Matched learning (M-learning) is a machine-learning technique that assesses how different people will respond to alternative treatments, especially when dealing with confounding factors. Instead of relying on inverse probability weighting, M-learning focuses on comparing well-matched pairs of patients to determine optimal treatment paths.
- Personalized Medicine: Moves away from the one-size-fits-all model, and it tailors treatments to individual characteristics.
- Individualized Treatment Rules (ITRs): Develops specific rules based on patient data to determine the most effective treatment strategies.
- Electronic Health Records (EHRs): Uses large-scale EHRs to gather comprehensive patient data, providing a base for personalized treatment plans.
- Machine Learning: Applies machine learning techniques to analyze EHRs and discover patterns for optimal treatment decisions.
- Observational Studies: Relies on observational data to understand real-world treatment effects, enhancing generalizability.
The Future of Diabetes Care: Data-Driven and Personalized
The application of matched learning to electronic health records is paving the way for a new era of personalized medicine. This advanced approach uses machine learning and AI to refine treatment strategies, improve results for type 2 diabetes patients, and also holds considerable promise for other fields of medicine. The move towards data-driven, personalized care represents a transformative step in improving healthcare outcomes and quality of life.