Can Robots Truly Understand and Boost Learning? The Rise of AI-Enhanced Education
"Explore how emotion and memory models in robots are revolutionizing mathematics education, offering personalized learning experiences and improved outcomes for students."
The integration of social robots in education is rapidly growing, particularly in how these robots can adapt to individual student needs. Recent studies highlight the importance of designing robots that understand and respond to user characteristics to enhance learning outcomes. Researchers are actively exploring machine learning methods to create adaptive behaviors in educational robots, with reinforcement learning algorithms gaining traction. These algorithms enable robots to learn and adjust their teaching strategies in real-time, significantly improving the effectiveness of tutoring compared to non-adaptive methods.
While many studies focus on implementing novel adaptation strategies in social robots, fewer explore their effectiveness in real-world settings within existing school curricula. Experts emphasize the necessity of conducting more in-the-field research to gain valuable insights into the future role of robots in education. Building on this need, recent work has developed emotion and memory models that enable robots to create memory accounts of children's emotional states. This advancement allows robots to tailor their behavior based on past interactions, enhancing long-term engagement and vocabulary learning.
This article delves into a pioneering study that applies an emotion and memory model to a social robot in a mathematics learning scenario. The primary aim is to assess the model's impact on children's engagement and learning in a real-life educational context. By implementing scenarios that closely resemble actual classroom activities, this research seeks to validate the effectiveness of adaptive robots in education beyond vocabulary learning. It also addresses a critical gap in understanding how robots can truly enhance learning in practical, curriculum-based settings.
Emotion and Memory Model for Personalized Learning
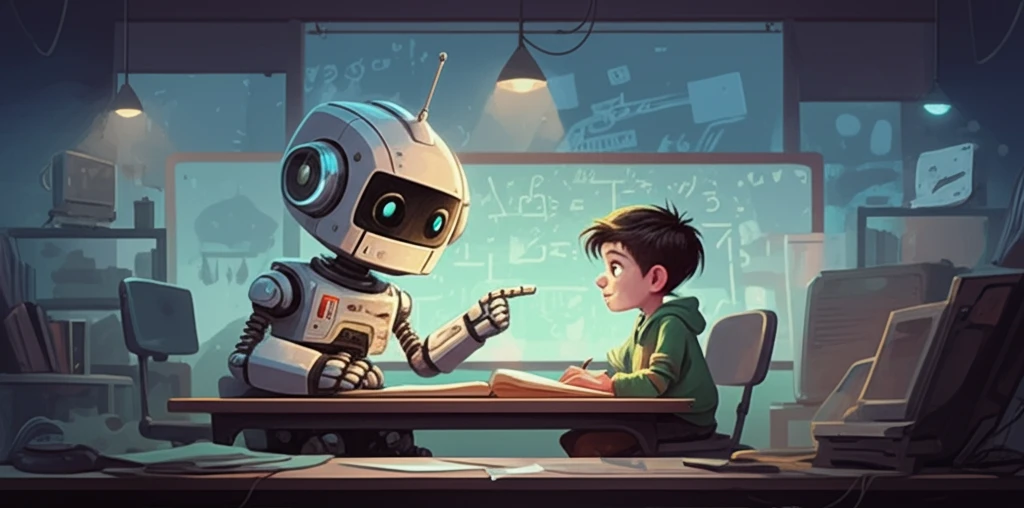
The emotion and memory model is designed to mimic how humans create and recall emotional memories. Humans store memories of both positive and negative events, categorizing them into conscious (explicit, declarative) and unconscious (implicit, procedural) memory systems. Emotional memory involves the implicit learning and storage of information about the emotional significance of events, while the memory of emotional situations refers to conscious memory. When emotional events occur, they are processed by the sensory system and transmitted to the temporal lobe or amygdala, forming either explicit or implicit memories. These memories are then retrieved when a related cue is encountered.
Future Directions
This study underscores the potential of integrating emotion and memory models into educational robots to enhance children's learning and social engagement. The findings suggest that robots equipped with these models can create more personalized and effective learning experiences. Future research should focus on evaluating these models in various real-life educational scenarios to further refine their impact on learning outcomes.