Breathe Easier: Unlocking the Secrets to Cleaner Air and Ozone Awareness
"Navigating air quality: Understand ozone signals amidst weather changes for a healthier environment."
In today's world, knowing about air quality is crucial, and this knowledge comes from the ability to spot different signals in the air, whether they are from weather, chemicals, or other sources. Figuring out these signals helps us understand how our climate is changing and how it affects the air we breathe.
Detecting changes in air quality is tricky because the air is always changing due to weather and natural events. Scientists use different ways to filter out this 'noise' to find real, important changes. Two common methods are looking at data over a long time (years) and averaging data from large areas. This helps in seeing past the everyday changes to find significant trends.
This article explores new research on how to best use these methods by looking at surface ozone, a key part of air quality. By understanding how weather affects ozone levels, we can make better recommendations for improving air quality predictions.
Maximizing Ozone Signals
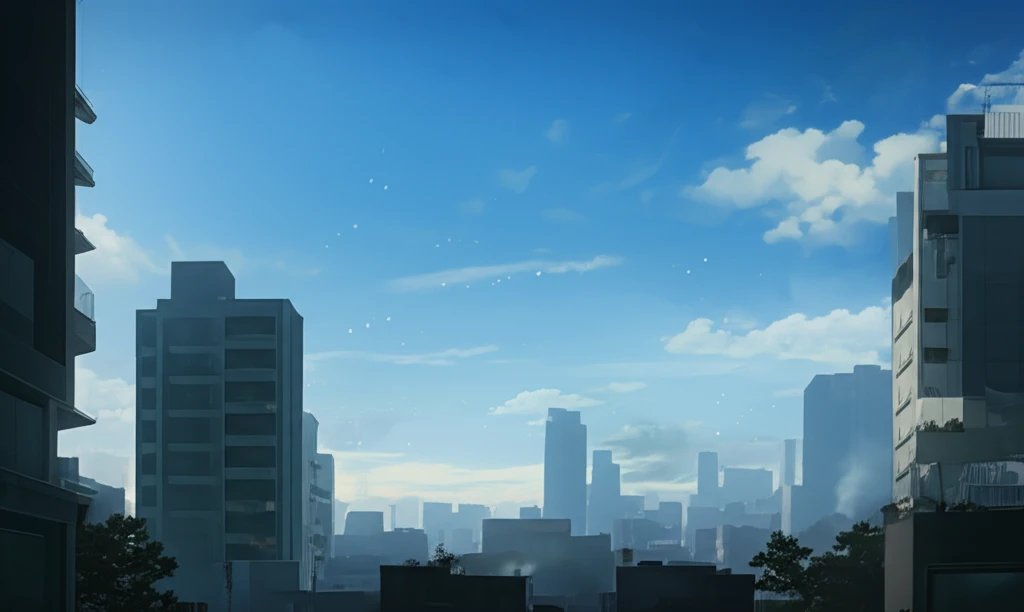
To accurately measure and analyze the signals in our atmosphere, research focuses on surface ozone to offer strategic methods for maximizing signal detection capabilities amidst chemical, meteorological, and climatological variability. Surface ozone serves as a key indicator, impacting both human health and environmental stability.
- Temporal Averaging: Analyzing data over extended periods to smooth out short-term fluctuations.
- Spatial Averaging: Collecting data from broad geographic areas to reduce local anomalies.
- Model Simulations: Using computer models to predict future ozone levels under various conditions.
- Trend Detection: Identifying long-term patterns in ozone concentrations to inform policy and mitigation strategies.
Moving Towards Clearer Skies
The road to cleaner air requires a mix of detailed research and smart use of data. By fine-tuning how we measure and analyze air quality, we can better tackle the problems of ozone pollution and its effects on our environment and health. This article supports the ongoing effort to improve our skies for future generations.