Beyond the Black Box: How "Cutting Feedback" Could Revolutionize Economic Forecasting
"A groundbreaking new approach to building economic models tackles uncertainty and delivers more reliable predictions. Learn how it could reshape our understanding of complex systems."
Economic forecasting is a notoriously tricky business. We rely on complex models to predict everything from GDP growth to inflation rates, informing critical decisions for businesses, governments, and individuals. But what happens when those models are wrong? Traditional economic models often treat different factors as separate components, but this approach can lead to significant inaccuracies if one part of the model is flawed or doesn't quite capture the real-world dynamics.
A new approach is emerging that directly tackles this problem: modular Bayesian inference with 'cutting feedback.' This innovative technique, outlined in a recent paper, offers a way to build more robust and reliable economic models by carefully controlling how different parts of the model influence each other. Think of it as a way to 'insulate' your predictions from the impact of potential errors or uncertainties in specific areas.
This isn't just an academic exercise. The paper demonstrates that this 'cutting feedback' approach can lead to significantly better economic forecasts, especially when dealing with complex, high-dimensional models. It could potentially reshape how economists and policymakers approach forecasting and risk assessment.
What is 'Cutting Feedback,' and Why Does It Matter?
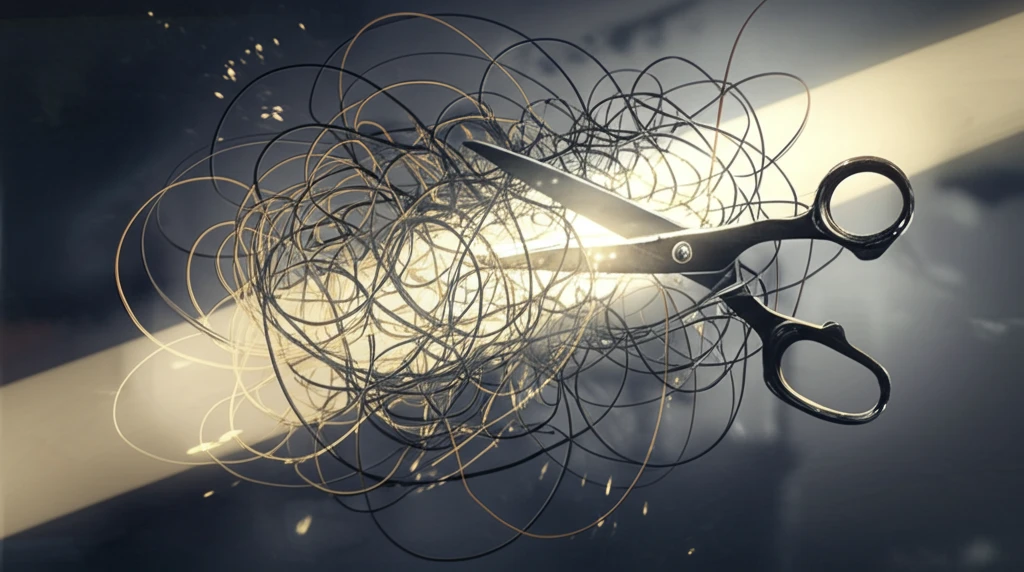
At its heart, 'cutting feedback' is about carefully managing the flow of information within a statistical model. Imagine you're building a model to predict the stock market. You might include factors like interest rates, inflation, and consumer confidence. In a traditional model, all these factors directly influence the final prediction. However, if your model of consumer confidence is flawed, it could throw off the entire forecast.
- Modular Bayesian Inference: Divides a complex model into smaller, manageable components or "modules."
- Feedback Loops: Recognizes that components in a model influence each other.
- Cutting Feedback: Limits the influence of potentially misspecified modules to improve overall accuracy.
The Future of Economic Modeling?
The 'cutting feedback' approach is still relatively new, but it holds significant promise for improving the accuracy and reliability of economic forecasting. By carefully managing the flow of information within complex models, economists and policymakers can potentially make more informed decisions and better navigate the uncertainties of the global economy. As the authors of the original paper conclude, further exploration of this Bayesian methodology could unlock even more useful applications in the world of economic modeling.