Beyond the Average: How Trimmed Means Offer a More Robust View of Data
"Tired of skewed data? Discover how trimmed means, a statistical technique, could provide a more balanced perspective on complex datasets and unlock hidden insights."
In a world awash with data, making sense of the numbers is more critical than ever. Traditional averages, like the mean, are often the go-to method for summarizing information. However, these averages can be easily skewed by extreme values, or outliers, leading to a distorted picture of the underlying trends. This is where trimmed means come in – offering a robust alternative that minimizes the impact of outliers and provides a more reliable representation of the data.
Think of it like this: imagine you're calculating the average income in a neighborhood, but one resident is a billionaire. That single outlier would drastically inflate the average, making it seem like everyone is much wealthier than they actually are. A trimmed mean, on the other hand, would remove the highest and lowest incomes before calculating the average, giving a more accurate reflection of the typical resident's financial situation.
This article delves into the world of trimmed means, exploring their applications, advantages, and how they can provide valuable insights in various fields. We'll unpack the statistical theory in an accessible way, showing you how to use this powerful tool to make better decisions and gain a deeper understanding of the data around you.
What are Trimmed Means and Why Should You Care?
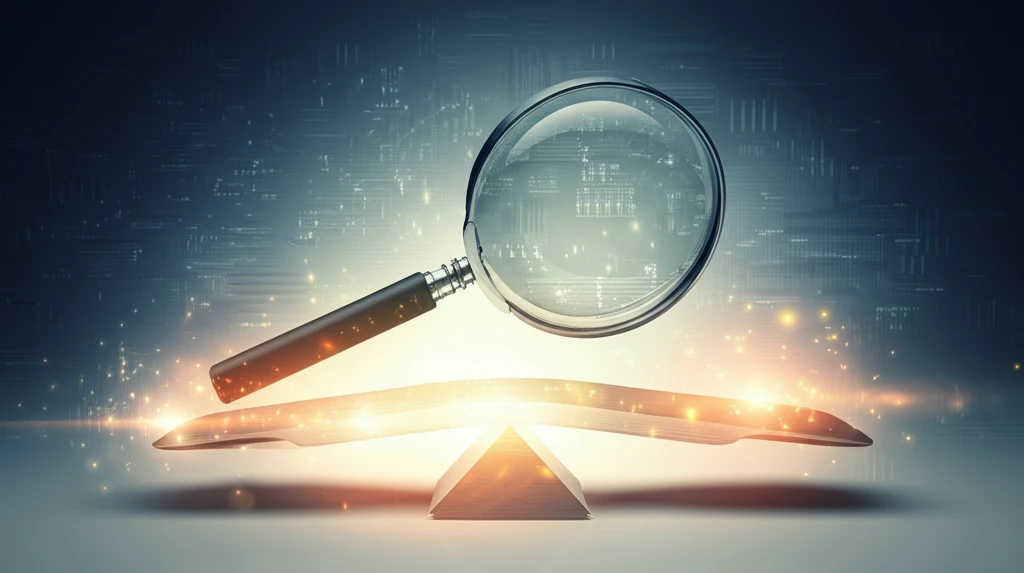
At its core, a trimmed mean is a modified average that excludes a certain percentage of the extreme values from a dataset before calculating the mean. This trimming process reduces the influence of outliers, providing a more stable and representative measure of central tendency. The 'trimming percentage' determines how much of the data is removed from each end of the distribution. For example, a 10% trimmed mean would remove the lowest 10% and the highest 10% of the values.
- Reduced Sensitivity to Outliers: The primary benefit is a more accurate representation of the 'typical' value, as extreme data points have less influence.
- Increased Stability: Trimmed means are less likely to fluctuate wildly due to the presence of a few unusual observations.
- Flexibility: The trimming percentage can be adjusted to suit the specific characteristics of the dataset and the research question.
- Applicability Across Disciplines: From finance to environmental science, trimmed means can be used in any field where data analysis is crucial.
Embrace the Power of Trimmed Means for Clearer Insights
In conclusion, trimmed means are a valuable tool for anyone working with data. By reducing the influence of outliers, they provide a more robust and reliable measure of central tendency, leading to clearer insights and better decision-making. So, the next time you're faced with a dataset that might be prone to extreme values, consider using a trimmed mean to unlock a more accurate and balanced perspective.