Beyond the Algorithm: Uncovering Hidden Food Pairings for a Healthier You
"Dive into the surprising science of food associations and how it can revolutionize dietary recommendations."
In a world saturated with diet fads and generic nutritional advice, it's easy to feel lost. Recommender systems promise personalized guidance, but often fall short due to reliance on extensive user data, which is not always available. Many people are looking for unique diet options and end up in dead end results. But what if the secret to better nutrition lay not in complex algorithms, but in the simple, often overlooked, relationships between the foods we eat every day?
Imagine a system that learns from the collective eating habits of a population, identifying common food pairings and suggesting complementary items to create more balanced and complete meals. This isn't just about recommending any food, but foods that are statistically more likely to be eaten together, reflecting underlying dietary patterns and preferences.
This approach moves beyond individual preferences and behaviors, tapping into a broader understanding of how people actually eat. By focusing on food associations, we can uncover hidden nutritional synergies and address the challenges of limited data and the ever-elusive 'cold-start' problem in personalized nutrition.
The Science of Food Associations
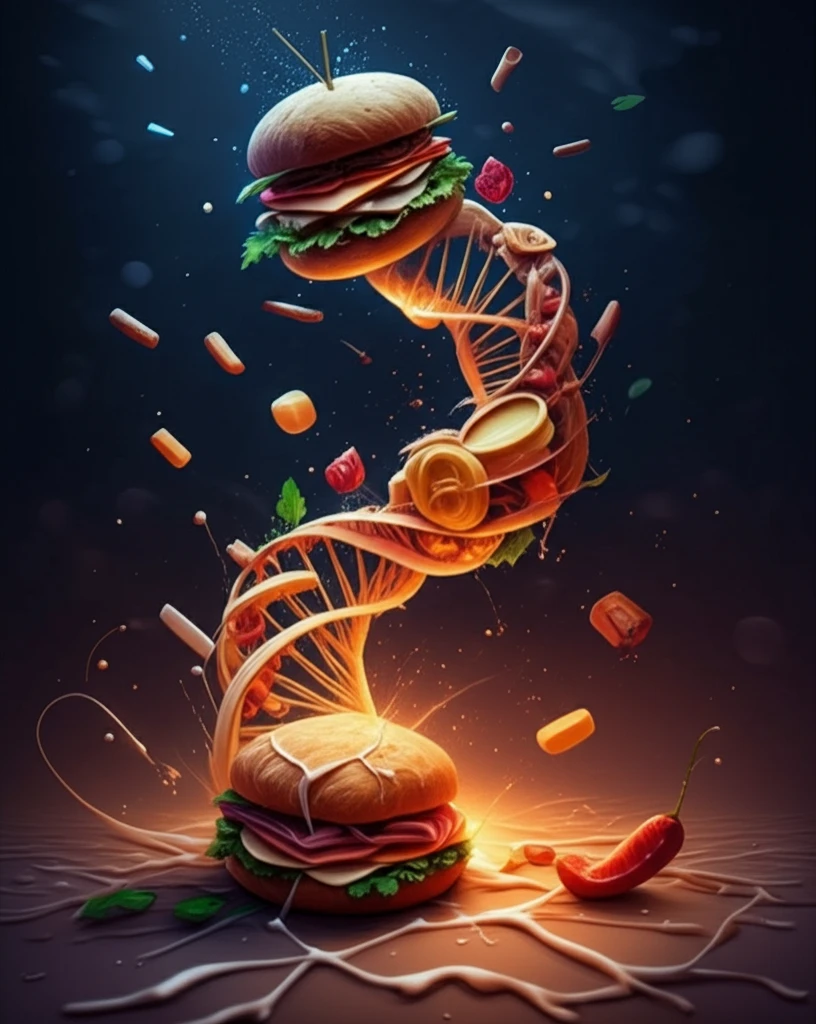
The challenge is that most recommender systems rely on individual user profiles and extensive histories of user choices. In the real world, people don't always have the luxury of time, or they might not want to share their data. That’s where food association techniques come into play, building a model from a population's aggregated dietary habits. This solves the problem of people not wanting to share data and people being too busy to find unique diet options.
- Data Mining: Food items that are consumed together are mined and discovered.
- Confidence Levels: Each association is assigned a confidence level, indicating how likely the second food is to be eaten given the first.
- Ontologies: Domain ontologies can be combined with food associations to get extract and formulate new knowledge.
- Algorithmic recommendations: Association rules need to be adapted for recommendation tasks, as exploratory data analysis may not require specificity.
The Future of Personalized Nutrition
By shifting the focus from individual profiles to collective eating behaviors, we can unlock new possibilities for personalized nutrition. This approach allows for the development of recommender systems that are more robust, adaptable, and relevant to the diverse needs of individuals seeking to improve their diets. The future of nutrition may lie not in complex algorithms, but in the simple, powerful connections between the foods we eat.