Beyond the Algorithm: How 'Stacking' AI Models Can Level the Playing Field in Economics and Social Sciences
"Discover how double machine learning (DDML) and model averaging are creating fairer, more reliable research outcomes, especially for underrepresented groups."
In the quest for unbiased insights, researchers are increasingly turning to machine learning to dissect complex datasets. Supervised machine learning estimators, prized for their ability to handle intricate relationships, are now pivotal in causal inference. Techniques like the post-double-selection lasso (PDS lasso) have risen to prominence, yet challenges persist. Recent studies reveal that reliance on single, pre-selected machine learning models can inadvertently skew results, particularly when dealing with the messy realities of economic data.
The core issue? Many machine learning methods hinge on assumptions like sparsity—the idea that only a few variables truly matter. When these assumptions fail to hold, or when the selected model doesn't quite fit the data's underlying structure, the resulting analysis can be misleading. This is where the concept of ‘stacking’ comes in, offering a powerful strategy to mitigate these risks.
This article explores how combining double or debiased machine learning (DDML) with stacking techniques can improve the robustness and reliability of research findings. By averaging multiple candidate learners, stacking reduces the dependence on any single model's assumptions, leading to more balanced and accurate outcomes. We'll delve into the mechanics of this approach, showcasing its potential to address biases and enhance the fairness of economic and social research.
The Power of Averaging: Stacking Explained
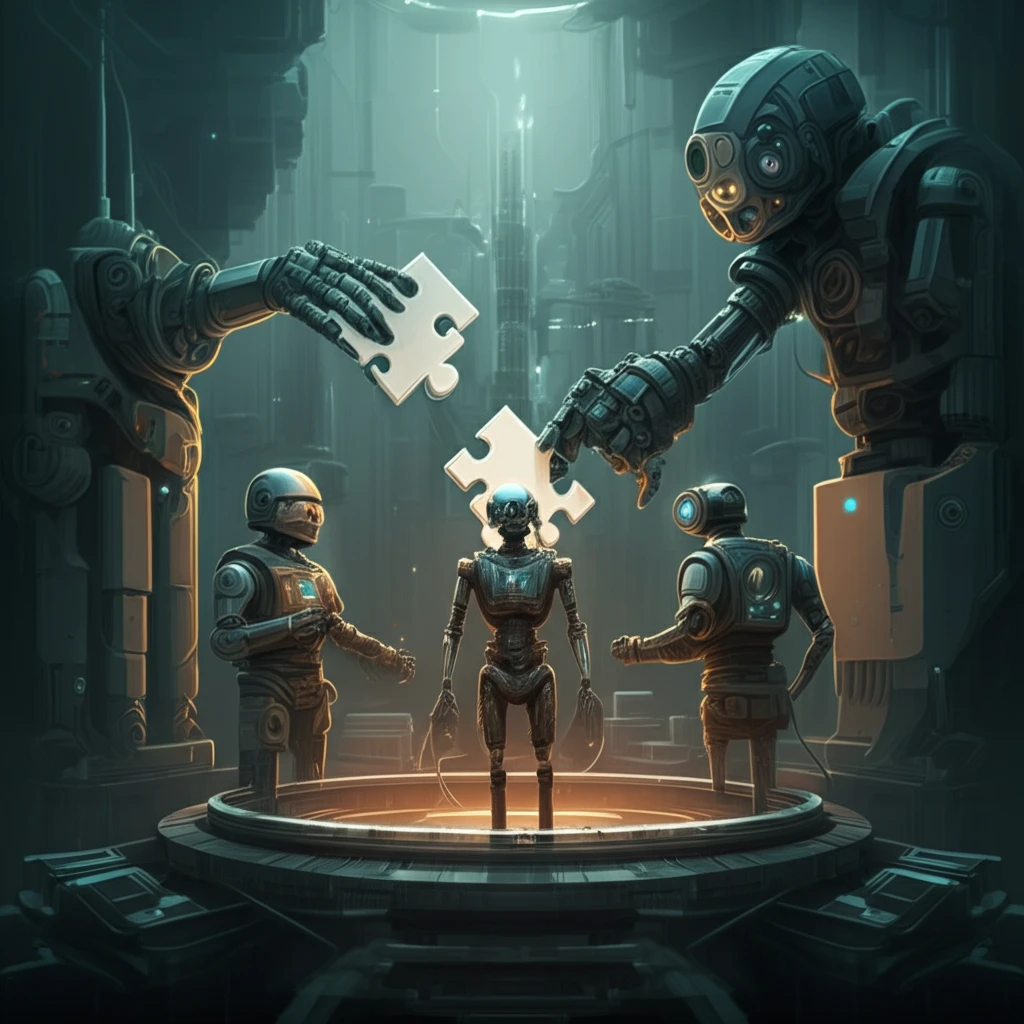
Stacking, at its heart, is a model averaging method. Instead of relying on one pre-selected machine learning algorithm, it combines multiple candidate learners to estimate structural parameters. Think of it as assembling a diverse team of experts, each with unique skills and perspectives, to tackle a complex problem. This approach is particularly valuable in situations where the ‘true’ relationships within the data are partially unknown.
- Short-stacking: Reduces the computational burden by leveraging the cross-fitting step inherent in DDML.
- Pooled stacking: Decreases variance by enforcing common stacking weights across cross-fitting folds. This enhances interpretability and stability.
Towards Fairer Insights
The synthesis of DDML with stacking represents a significant step forward in the pursuit of robust and equitable research. By mitigating the risks associated with single-model reliance, stacking promotes more reliable and transparent outcomes, particularly when examining sensitive topics like gender disparities. As machine learning continues to permeate various fields, these advanced techniques will be essential for ensuring the insights we gain are both accurate and fair.