Beyond Shape: How Persistence Homology is Changing Data Analysis
"Unlocking New Dimensions in Data Science with Topology"
In an era drowning in data, finding meaningful patterns and insights is like searching for a needle in a haystack. Traditional data analysis often focuses on geometric shapes and statistical summaries, but what if the most important information lies hidden in the underlying structure of the data itself? This is where persistence homology steps in, offering a powerful new way to see the invisible.
Persistence homology is a technique that extracts topological features – things like connected components, holes, and voids – that persist across different scales of resolution. Imagine analyzing a network of social connections. Traditional methods might focus on the most popular individuals or the average number of connections. Persistence homology, on the other hand, can identify tightly-knit communities, influential bridges between groups, and even hierarchical relationships within the network. These topological insights can reveal patterns that would otherwise remain hidden.
While the math behind persistence homology can seem intimidating, the core idea is surprisingly intuitive, and its applications are rapidly expanding across diverse fields. We'll explore how this tool works, why it's so valuable, and where it's making the biggest impact.
The Power of Seeing Beyond Shape
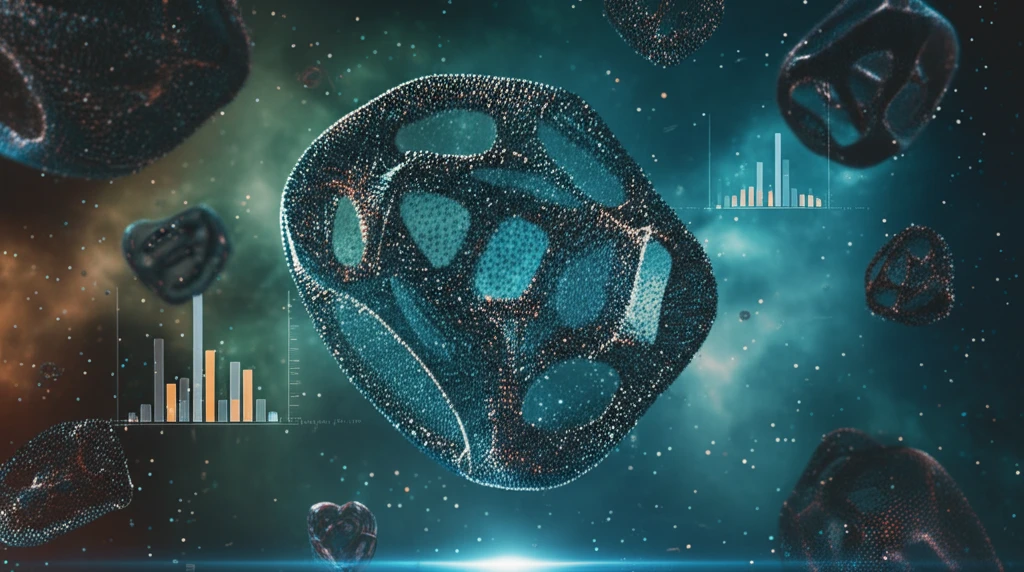
At its heart, persistence homology is about understanding how topological features appear and disappear as we "zoom in" or "zoom out" on a dataset. Think of it like exploring a mountain range. From a distance, you might only see a few major peaks. As you get closer, smaller peaks and valleys become visible, and the connections between them start to emerge. Persistence homology captures this process systematically, tracking the birth and death of topological features at different scales.
- Build a Filtration: Create a series of nested shapes (a filtration) from your data, typically by connecting points that are close together at increasing distances.
- Track Topological Features: As the shapes grow, identify when new connected components, holes, or voids appear (birth) and when they disappear (death).
- Create a Persistence Diagram: Plot the birth and death times of each feature on a diagram. Features that persist for a long time will be far from the diagonal.
- Interpret the Results: Analyze the persistence diagram to identify the most significant topological features and extract meaningful insights about your data.
The Future is Topological
Persistence homology is still a relatively young field, but its potential is undeniable. As data continues to grow in size and complexity, techniques like persistence homology will become increasingly essential for extracting meaningful insights and making better decisions. Whether it's discovering new drug targets, designing more resilient materials, or understanding the complexities of social networks, persistence homology offers a powerful new lens for seeing the world around us.