Balancing the Equation: How a New Method Fine-Tunes Regression Analysis
"Discover how Empirical Likelihood Covariate Adjustment enhances precision in regression discontinuity designs, offering sharper insights for researchers and policymakers."
In the world of number crunching, getting the most accurate results from data is a constant challenge. Regression analysis, a cornerstone of research in economics, social sciences, and beyond, often grapples with biases and uncertainties that can muddy the waters. One particular area, regression discontinuity (RD) designs, seeks to pinpoint cause-and-effect relationships by examining sharp cutoffs, like eligibility thresholds for a program. But what happens when these analyses are thrown off by other factors at play?
Enter Empirical Likelihood Covariate Adjustment, a method designed to address these very issues. Imagine trying to determine the impact of a scholarship on college enrollment, but realizing that family income, prior academic performance, and access to resources all influence the outcome. This new method acts like a highly skilled filter, carefully balancing these 'covariates' to provide a clearer picture of the scholarship's true effect.
This approach, which directly incorporates covariate balance, has broad implications for anyone relying on regression analysis. By minimizing distortions and maximizing the use of available data, it promises to enhance the validity and reliability of research findings, ultimately leading to better-informed decisions and policies. Let's unpack this powerful technique and see how it's changing the landscape.
What is Empirical Likelihood Covariate Adjustment?
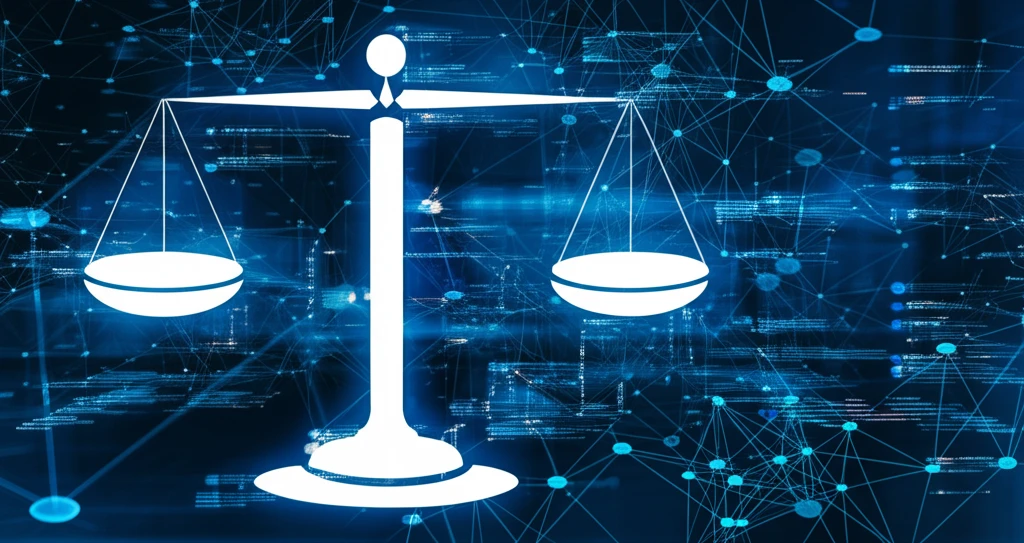
At its core, Empirical Likelihood Covariate Adjustment is a statistical technique designed to improve the precision of regression estimates by accounting for the influence of other variables (covariates). It's particularly useful in regression discontinuity (RD) designs, where researchers aim to isolate the impact of a specific intervention or treatment by examining outcomes around a critical threshold.
- Enhanced Precision: Reduces bias and provides more accurate estimates of the treatment effect.
- Flexibility: Can be applied to various RD-related settings and adapted to different types of covariates.
- Robustness: More resilient to slight deviations from the ideal covariate balance, making it suitable for real-world data.
The Future of Regression Analysis
Empirical Likelihood Covariate Adjustment represents a significant step forward in the quest for more accurate and reliable regression analysis. By directly addressing the challenge of covariate imbalance, it provides researchers and policymakers with a powerful tool for understanding cause-and-effect relationships. As data analysis continues to play an ever-greater role in shaping our world, methods like this will be essential for ensuring that decisions are based on the soundest possible evidence.