Are Government Benefits Boosting the Economy or Overstating Impact? What You Need to Know
"Uncover how underreported and imputed data in government programs could be skewing economic impact assessments, affecting everything from social security to child benefits."
In today's economy, government benefits play a crucial role, influencing everything from individual household stability to broader economic trends. Vast amounts of research are dedicated to understanding the impacts of these benefits, often relying on complex methods like instrumental variables (IV) to assess their effectiveness. However, the accuracy of these assessments hinges on the quality of the data used. What happens when the data isn't perfect?
A significant challenge arises from the fact that household surveys, a primary source of data on government benefits, are often plagued by inaccuracies. Benefits may be underreported, understated, or based on imputed values—estimates used when actual data is missing. This is not just a minor statistical problem; it can fundamentally distort our understanding of how these programs truly affect people and the economy.
This article explores how these data imperfections can lead to inflated estimates of program impacts, drawing on economic research that dives deep into the issue. By understanding these biases, individuals can gain a more realistic perspective on the true effects of government programs, helping them make more informed decisions.
The Hidden Pitfalls: How Data Errors Skew Economic Impact
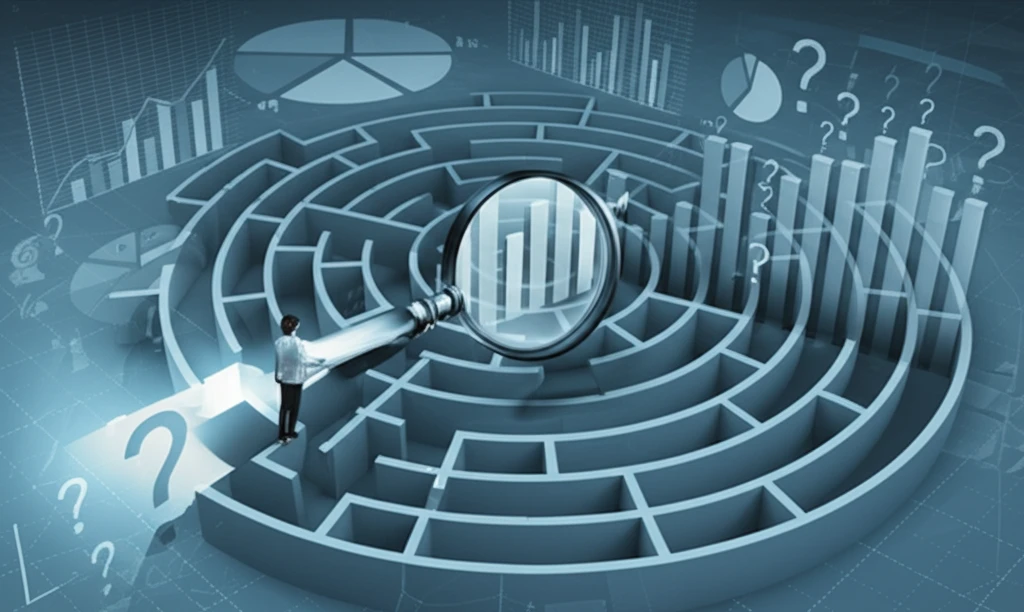
Economic studies frequently use instrumental variables (IV) methods to evaluate government benefits, treating benefit amounts as factors in economic analysis. The problem is that the data collected from household surveys, which forms the backbone of these evaluations, isn't always accurate. A significant portion of this data includes underreported figures or imputed values, which are essentially educated guesses when real numbers are missing.
- Underreporting: Individuals might not accurately report the benefits they receive, either due to memory lapses or privacy concerns.
- Imputation: When data is missing, statistical models are used to estimate the missing values. These models, however, are not always perfect and can introduce bias.
- IV Methods: Instrumental variables methods rely on the assumption that the instruments used are not correlated with the error term in the regression. However, when the dependent variable is measured with error, this assumption may be violated.
Navigating the Data Maze: Steps Toward More Accurate Assessments
The issue of data accuracy in assessing government benefit programs is complex. It requires careful attention from researchers and policymakers alike. By acknowledging and addressing these challenges, it’s possible to move toward more reliable and realistic evaluations of these crucial programs, ensuring that policies are based on solid evidence rather than skewed perceptions.