AI in the Courtroom: Can Algorithms Truly Judge Better Than Humans?
"A new study digs deep into whether AI helps human judges make more accurate decisions, finding surprising results about risk assessment tools and the future of justice."
Artificial Intelligence (AI) is rapidly transforming numerous aspects of our lives, and the courtroom is no exception. Data-driven algorithms are increasingly being used to aid in judicial decisions, from assessing the risk of releasing defendants on bail to predicting the likelihood of recidivism. Yet, even with these technological advancements, human judges remain the final arbiters in most legal cases. This begs the critical question: Does AI truly help humans make better decisions in the justice system, or are we placing undue faith in the power of algorithms?
Recent research has largely focused on whether AI recommendations are accurate or biased. However, a groundbreaking study introduces a new framework for evaluating AI's impact on human decision-making in experimental and observational settings. This innovative methodology seeks to determine whether AI recommendations genuinely improve a judge's ability to make correct decisions, compared to scenarios where judges rely solely on their own judgment or systems relying entirely on AI.
This analysis bypasses the problems of selective labels, addressing how endogenous decisions impact potential outcomes. By focusing on single-blinded treatment assignments, the study offers a rigorous comparison of human-alone, human-with-AI, and AI-alone decision-making systems. The results? They might just challenge your assumptions about the role of AI in the pursuit of justice.
Decoding the Methodology: How Can We Evaluate AI in the Courtroom?
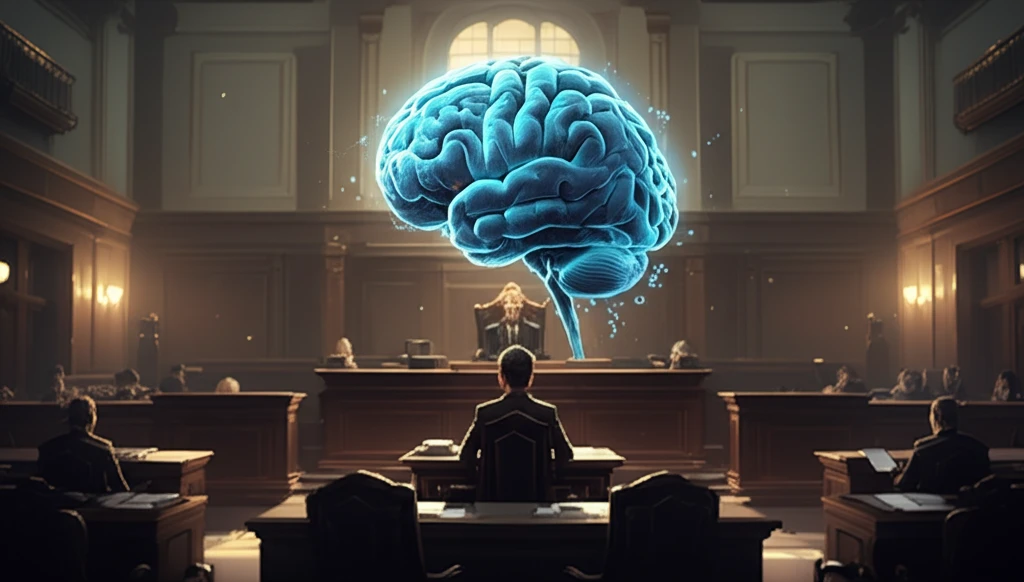
The study introduces a robust methodological framework designed to evaluate the statistical performance of human-alone, human-with-AI, and AI-alone decision-making systems. The framework begins with a few core assumptions. The study considers a single-blinded treatment assignment, ensuring that only human decisions—not direct interactions with AI—affect an individual’s outcome. It also assumes that the AI recommendations are randomized across cases, at least conditionally on observed covariates.
- Single-Blinded Treatment Assignment: AI recommendations influence outcomes solely through human decisions.
- Unconfounded Treatment Assignment: Assignment of AI is independent of potential outcomes, given pre-treatment covariates.
- Overlap: Each case has a non-zero probability of receiving AI recommendations.
Looking Ahead: The Future of AI in Judicial Decision-Making
The integration of AI into judicial decision-making is still in its early stages, and many questions remain about its optimal role. As AI technology continues to evolve, ongoing research and rigorous evaluation will be crucial to ensure that these tools are used responsibly and ethically. By carefully considering the potential benefits and limitations of AI, we can work towards a more just and equitable legal system for all.