AI Decision-Making: Can Algorithms and Humans Truly Collaborate?
"Explore the pitfalls and potential of combining AI predictions with human judgment for better decisions."
In today's world, decisions are more complex than ever. To help, algorithms are being used in important areas like criminal justice, healthcare, and lending. These algorithms analyze data and make predictions, which are then used to make decisions either automatically or with the help of human agents.
While these human agents may have valuable knowledge, they can also have biases or limitations that don't line up with the algorithm. Take, for instance, a child protection service using an algorithm to assess risk. A social worker might have useful intuition, but also personal biases that affect their judgment. This raises the question: How should we design these algorithms to work effectively with human decision-makers?
New research offers insights into this challenge, revealing how to design algorithms and delegate decisions for better outcomes. The findings suggest that simply providing more information isn't always the best approach, and that policies like always having a 'human-in-the-loop' can sometimes make things worse. By understanding these dynamics, we can create more effective collaborations between humans and AI.
The Delicate Balance: When Should AI Decisions Be Handled by Humans?
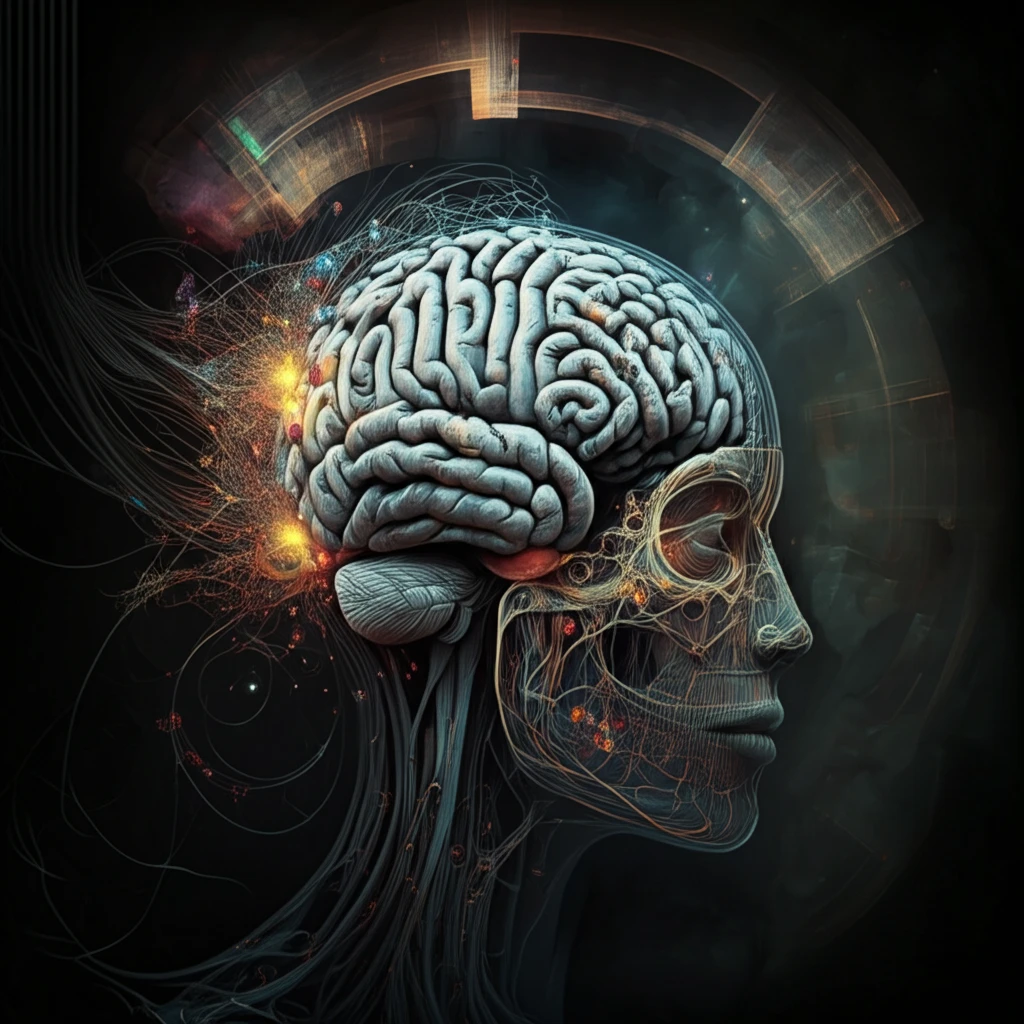
The study models a scenario where a principal (like a manager or policymaker) designs an algorithm to predict a binary outcome (like whether a loan will be repaid or a patient will respond to treatment). The principal must then decide whether to act directly on the algorithm's prediction or delegate the decision to an agent who has private information but may be misaligned with the principal's goals.
- Delegation Improves Outcomes If: The principal would make the same decision as the agent with the agent's information.
- Misalignment Risks: Delegation can be counterproductive if the agent's interests diverge from the principal's.
- Understanding Human Factors: Incentives and potential biases of human decision-makers are crucial when designing AI systems.
Avoiding the Pitfalls: Toward Better Human-AI Collaboration
The study cautions that well-intentioned policies, such as always including a human in the decision-making loop or aiming for maximum prediction accuracy, can sometimes worsen the quality of decisions. This is particularly true when there's a misalignment between the algorithm's goals and the human agent's incentives. The findings offer a possible explanation for why human-machine collaborations often underperform in real-world scenarios. By understanding these potential pitfalls and carefully designing algorithms and delegation strategies, we can harness the power of AI to make better, more informed decisions.