A/B Testing Evolved: How Geometric Mixing Overcomes Switchback Experiment Pitfalls
"Unlock reliable insights with geometric mixing techniques to optimize online marketplaces and beyond, avoiding the hidden biases of traditional switchback experiments."
In the dynamic world of online marketplaces and data-driven decision-making, A/B testing reigns supreme. Businesses are constantly experimenting with new features, pricing models, and user interfaces, all in the pursuit of optimizing performance and enhancing user experience. Among the various A/B testing methodologies, switchback experiments have emerged as a popular tool, particularly when dealing with network effects and spillover between different experimental units.
Switchback experiments involve repeatedly toggling a treatment or intervention on and off for an entire system, such as a specific geographic market or a segment of users. This approach helps to mitigate biases caused by cross-unit interference, where the behavior of one unit influences others. Think of a new pricing scheme in a ride-sharing app: a switchback test can prevent market distortions that might arise if different pricing strategies were simultaneously deployed in separate regions.
However, switchback experiments are not without their own set of challenges. One of the most significant is the problem of temporal carryover effects, also known as lag effects. The impact of a treatment applied at a specific time may not be limited to that immediate period; it can linger and influence outcomes in subsequent periods. Ignoring these carryover effects can lead to biased results and flawed conclusions. This article delves into the intricacies of switchback experiments, exploring how a concept called "geometric mixing" can help overcome the limitations of traditional approaches and provide more reliable insights.
The Hidden Bias: Understanding Temporal Carryover Effects
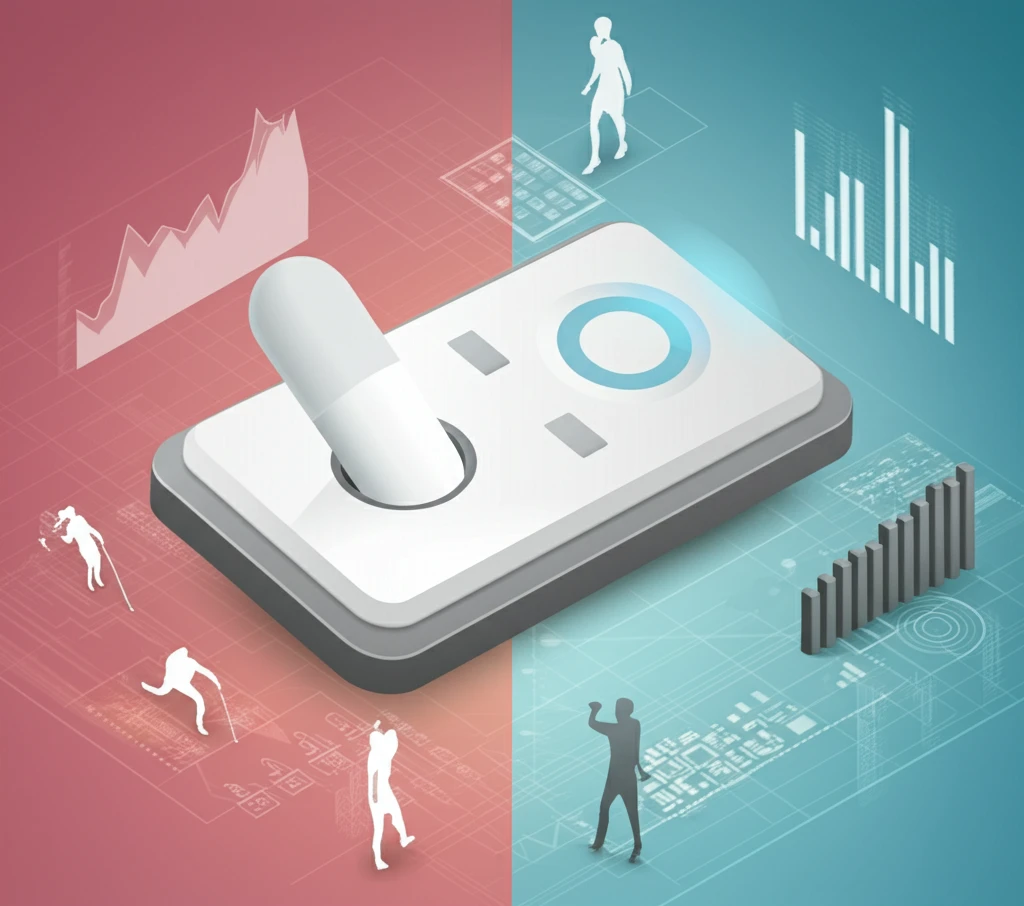
Temporal carryover effects arise because systems often possess a 'memory' – their current state is influenced by past events and actions. Consider an online marketplace implementing a new algorithm for matching jobs to available workers. If the new algorithm prioritizes long-term resource allocation over immediate needs, it might initially cause a temporary dip in performance for a 'greedy' matching strategy used as a control, or vice versa. This is because the system needs time to adjust to the new allocation patterns. Traditional switchback analyses, which simply compare average outcomes during 'on' and 'off' periods, may misattribute these initial fluctuations to the treatment itself, leading to inaccurate estimations of its true impact.
- Example: Imagine a switchback experiment testing a new website design. If users are initially confused by the new design, they might abandon their shopping carts. Even after switching back to the old design, some users may not return, leading to an underestimation of the new design's long-term potential if you don't account for lag.
- Impact on Decision-Making: If carryover effects aren't addressed, businesses might prematurely abandon promising interventions or, conversely, adopt changes that ultimately prove detrimental. This can lead to wasted resources, missed opportunities, and suboptimal performance.
- Real-world Scenarios: Carryover effects are prevalent in various domains, including marketing campaigns (where brand awareness lingers), healthcare interventions (where treatment effects persist), and educational programs (where learned skills endure).
Geometric Mixing: A Path to More Reliable A/B Testing
Switchback experiments are a powerful tool for data-driven decision-making, but their vulnerability to temporal carryover effects cannot be ignored. Geometric mixing offers a sophisticated framework for addressing this challenge, enabling businesses to unlock more reliable insights and optimize their strategies with greater confidence. By accounting for the dynamic nature of systems and the lingering effects of interventions, geometric mixing paves the way for more informed experimentation and ultimately, better outcomes.