Unlocking the Secrets of Statistical Distributions: A Modern Approach
"Discover how a new family of statistical distributions can enhance data analysis and predictive modeling, transforming complex data into actionable insights."
In the realm of statistics, distributions are fundamental tools for understanding and modeling data. From predicting stock prices to assessing public health risks, statistical distributions provide a framework for making informed decisions. However, traditional distributions sometimes fall short when dealing with complex, real-world data.
Recent research has focused on developing new families of distributions that can better capture the nuances of diverse datasets. One promising approach involves using order statistics—the values of a dataset arranged in ascending order—to construct novel probability distributions. This method offers greater flexibility and precision in modeling various phenomena.
This article delves into a cutting-edge study that introduces a new family of distributions derived from the probability density function (pdf) of order statistics. We’ll explore the methodology behind this innovative approach, examine its potential applications, and discuss its implications for data analysis and beyond. Get ready to unlock the secrets of statistical distributions and discover how they can transform complex data into actionable insights.
What are Order Statistics and Why Do They Matter?
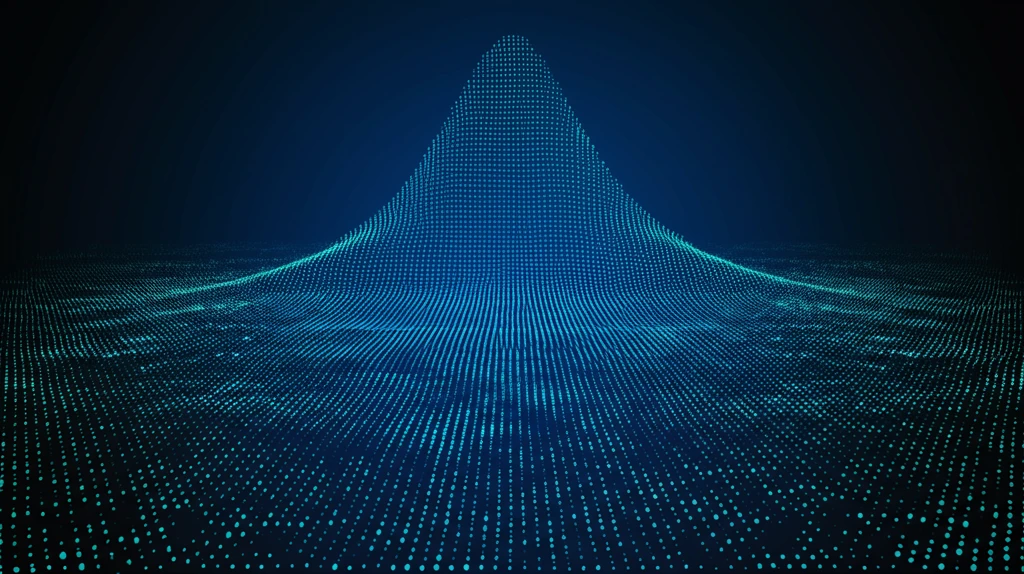
Order statistics, at their core, involve arranging a set of data points in ascending order. Imagine you have a collection of exam scores. Order statistics would sort these scores from lowest to highest, allowing you to easily identify the minimum, maximum, and median values. These sorted values provide valuable insights into the distribution and characteristics of the data.
- Flexibility: Order statistics allow for the creation of distributions tailored to specific datasets.
- Precision: These distributions can more accurately model complex phenomena.
- Adaptability: Suitable for non-identical and independent data points.
- Real-World Applications: Useful in diverse fields such as finance, healthcare, and environmental science.
The Future of Statistical Modeling
The development of new families of distributions using order statistics represents a significant advancement in statistical modeling. By providing greater flexibility and precision, these methods empower researchers and analysts to gain deeper insights from complex data. As data continues to grow in volume and complexity, the importance of innovative statistical tools will only increase. Embrace the power of order statistics and unlock new possibilities in data analysis.