Smart Farming: Can AI Level the Playing Field for Sustainable Agriculture?
"Discover how artificial intelligence and innovative algorithms are revolutionizing land leveling, paving the way for more efficient and eco-friendly farming practices."
As the global population surges, the demand for agricultural commodities is reaching unprecedented levels. This escalating need underscores one of today's most pressing environmental challenges: optimizing energy production and consumption in agriculture. While modern solutions like solar energy offer promise, the reality is that inefficient practices and inadequate management continue to drive up energy use within the sector.
Among the various agricultural operations, land leveling stands out as particularly energy-intensive and costly. The process not only consumes significant amounts of power but also risks compacting the soil, especially in wetter conditions. Such compaction can lead to long-term damage that is difficult to reverse, hindering agricultural productivity. However, land leveling is essential as it streamlines irrigation, improves field conditions, and normalizes soil surfaces, directly impacting crop yields.
Fortunately, innovations are emerging to address these challenges. Researchers are increasingly turning to artificial intelligence (AI) and machine learning to optimize energy consumption during land leveling. One promising approach involves using Artificial Neural Networks (ANNs) in conjunction with Imperialist Competitive Algorithms (ICA), creating a smarter, more efficient method for land preparation. This blend of technology aims to minimize environmental impact while maximizing agricultural output.
How AI and ICAs are Transforming Land Leveling
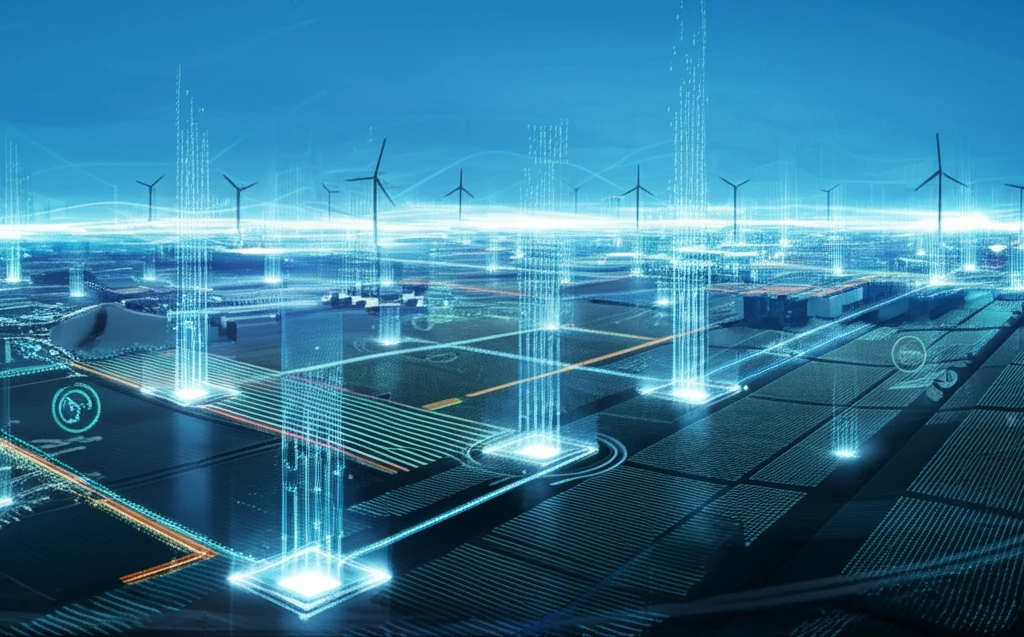
The integration of AI, particularly through ANNs, offers a sophisticated way to model and predict outcomes in complex systems. ANNs can analyze numerous variables to determine the most energy-efficient strategies for land leveling. This approach moves beyond traditional methods that often overlook critical factors affecting energy consumption.
- Data Collection: Detailed data on soil properties, including density, moisture content, and slope, are gathered.
- ANN Training: The ANN is trained using this data to predict energy consumption under various conditions.
- ICA Optimization: The ICA optimizes the ANN by adjusting parameters to minimize energy use and cost.
- Sensitivity Analysis: A sensitivity analysis identifies which factors most significantly impact energy consumption, allowing for targeted adjustments.
The Future of Farming is Smart
As we face increasing demands on our agricultural systems, embracing AI-driven solutions like ANN-ICA models is no longer just an option but a necessity. By optimizing energy consumption, reducing environmental impact, and enhancing crop yields, we pave the way for a more sustainable and efficient future for farming. This technological integration promises not only to improve agricultural practices but also to secure our food supply for generations to come.