Decoding Your Home's Energy Story: How Hidden Markov Models Reveal Hidden Thermal Patterns
"Unlocking energy efficiency: A data-driven approach to understanding household energy use and thermal behavior through Hidden Markov Models."
In an era where sustainable living is no longer a trend but a necessity, understanding our energy consumption patterns is crucial. With heating and cooling accounting for a significant portion of household energy use, finding ways to optimize this consumption without sacrificing comfort is a key challenge. While many solutions focus on improving the efficiency of building spaces and employing scalable technologies, the unique operational characteristics of each home often get overlooked.
Imagine being able to 'listen' to your home, not through traditional sensors and meters alone, but by interpreting the subtle language of its energy use. This is where data-driven models, particularly those leveraging Hidden Markov Models (HMMs), come into play. HMMs offer a unique lens through which to view energy consumption, revealing underlying patterns and hidden states that traditional methods might miss. By understanding these hidden thermal profiles, we can create more flexible and responsive energy management systems.
This article delves into the world of HMMs and their application in decoding household thermal behavior. We'll explore how these models transform readily available smart meter data into actionable insights, providing a pathway to reduce energy consumption while maintaining optimal comfort levels. Join us as we unravel the mysteries of your home's energy story and discover how HMMs can lead to a more sustainable and efficient future.
What are Hidden Markov Models (HMMs) and How Do They Work?
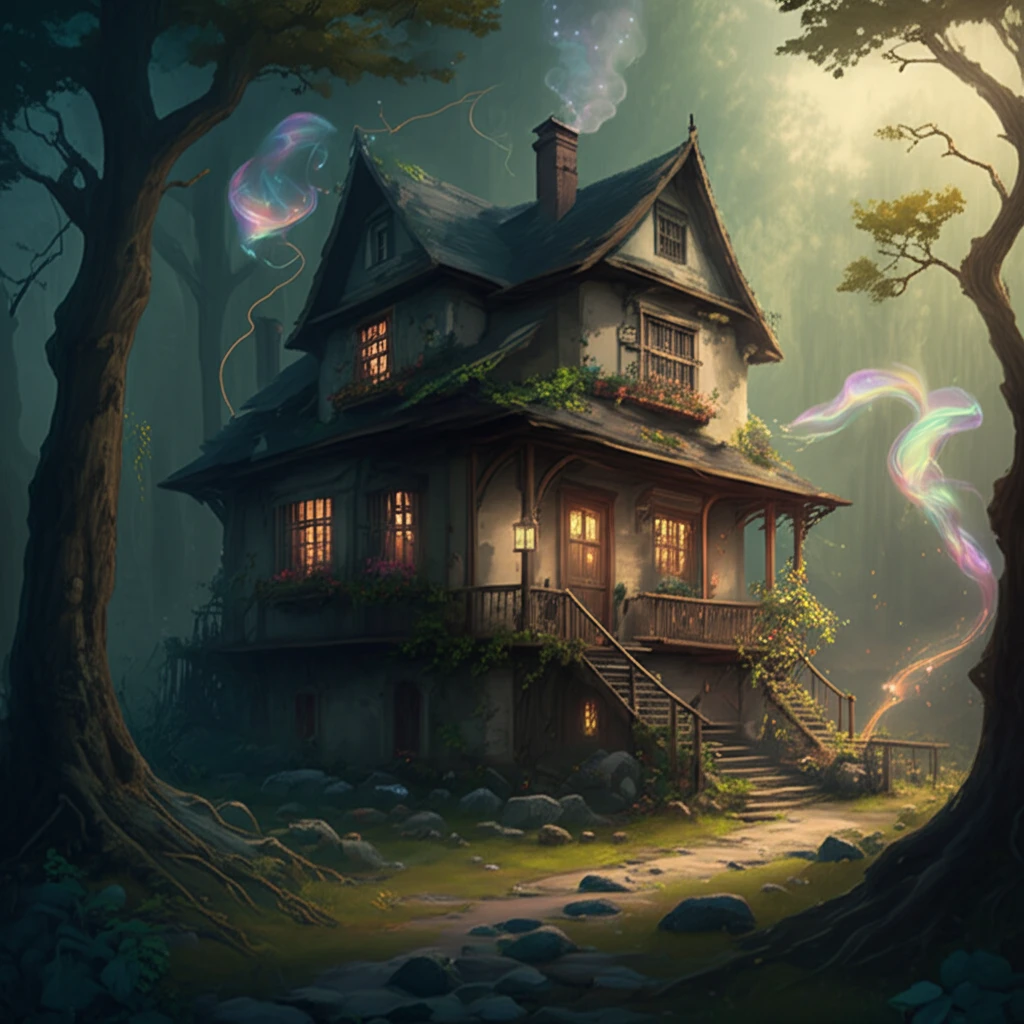
Hidden Markov Models (HMMs) are statistical models used to represent systems that evolve through a series of unobserved (hidden) states. The power of HMMs lies in their ability to infer these hidden states from a sequence of observed data. Think of it like this: imagine a machine that performs different tasks (the hidden states), but you can only see the results of these tasks, not the tasks themselves. An HMM helps you deduce what tasks the machine was performing based on the observed outcomes.
- States: The different, unobservable conditions or modes of the system. In our case, thermal load levels (heating, cooling, equilibrium).
- Observations: The data we can directly measure. For example, hourly energy consumption and outdoor temperature.
- Transition Probabilities: The likelihood of moving from one state to another. For instance, the probability of transitioning from a 'high heating' state to a 'low heating' state.
- Emission Probabilities: The likelihood of observing a particular data point (energy consumption, temperature) given a specific state.
The Future of Home Energy Management with HMMs
The application of HMMs to household energy analysis opens exciting possibilities for the future of smart homes and energy management. By uncovering the hidden thermal patterns within our homes, we can develop more personalized and effective strategies for energy conservation. From optimizing HVAC systems to predicting energy demand and detecting anomalies, HMMs are poised to play a central role in creating a more sustainable and comfortable living environment.