Decoding the Secrets of Point Processes: How Repulsion Shapes the World Around Us
"Explore the fascinating world of determinantal point processes (DPPs) and how understanding repulsiveness can unlock new insights in fields ranging from telecommunications to ecology."
Have you ever noticed how trees in a forest often seem to maintain a certain distance from each other, or how Wi-Fi hotspots in a city aren't usually clustered too closely together? These patterns aren't random; they're often the result of 'repulsive point processes' at work. These processes describe situations where individual points or entities tend to spread out rather than clump together.
Determinantal point processes (DPPs) are a powerful class of mathematical models used to describe these kinds of repulsive patterns. In essence, DPPs help us understand and quantify how much 'personal space' different elements in a system try to maintain. This has practical implications in numerous fields, from designing efficient telecommunications networks to understanding biodiversity in ecological systems.
While the math behind DPPs can get complex, the core idea is surprisingly intuitive: DPPs provide a way to predict the likelihood of finding points close to each other based on how much repulsion exists between them. This article breaks down the key concepts of DPPs, explores their applications, and explains how researchers are working to refine these models to better reflect the real world.
What Exactly Are Repulsive Point Processes?
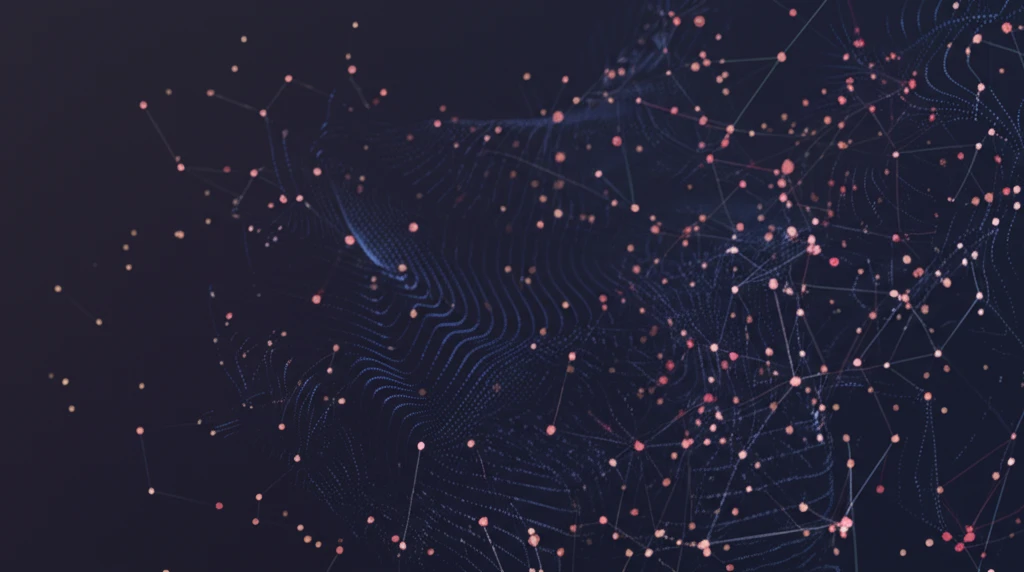
At their heart, repulsive point processes are about modeling spatial patterns where elements tend to avoid each other. Think of it like trying to seat guests at a dinner party – you wouldn't want to cram everyone together, you'd naturally spread them out to give each person some elbow room. In technical terms, these processes are used to model the probability of finding points (which could represent anything from trees to cell towers) at certain locations, taking into account the tendency of those points to repel each other.
- Spatial Statistics: Analyzing the distribution of crimes, diseases, or natural resources.
- Statistical Learning: Creating diverse and representative datasets for training machine learning models.
- Telecommunications: Optimizing the placement of network nodes to ensure coverage and minimize interference.
- Ecology: Studying the distribution of plant and animal species in an ecosystem.
The Future of DPPs: New Models and Applications
As our ability to collect and analyze spatial data continues to grow, DPPs are poised to play an even greater role in helping us understand the world around us. Ongoing research is focused on developing new DPP models that can capture even more complex types of repulsive interactions, as well as on finding efficient ways to apply these models to large datasets. From predicting the spread of diseases to designing smarter cities, the potential applications of DPPs are vast and exciting.