Decoding Sentiment: How AI Learns to Understand Your Online Mood
"Explore how AI is mastering sentiment analysis to bridge the gap between understanding human emotion and leveraging it across diverse online platforms and contexts."
In today's digital age, the internet pulses with human sentiment. Every tweet, review, and blog post is a snapshot of how someone feels, creating a vast and ever-changing landscape of opinions. But how can we make sense of this sea of emotions? Enter sentiment analysis, a field dedicated to automatically identifying and categorizing these feelings.
Sentiment analysis has become increasingly vital, and recent developments in Artificial Intelligence (AI) are revolutionizing this field. No longer are we limited to simple positive, negative, or neutral labels. AI algorithms are now capable of detecting sarcasm, understanding context, and even predicting the intensity of emotion behind the words.
This article dives deep into how AI is transforming sentiment analysis. We'll explore the challenges, the cutting-edge techniques, and the potential for this technology to shape everything from customer service to political campaigns.
The Challenge: Bridging the Gap Between Words and Feelings
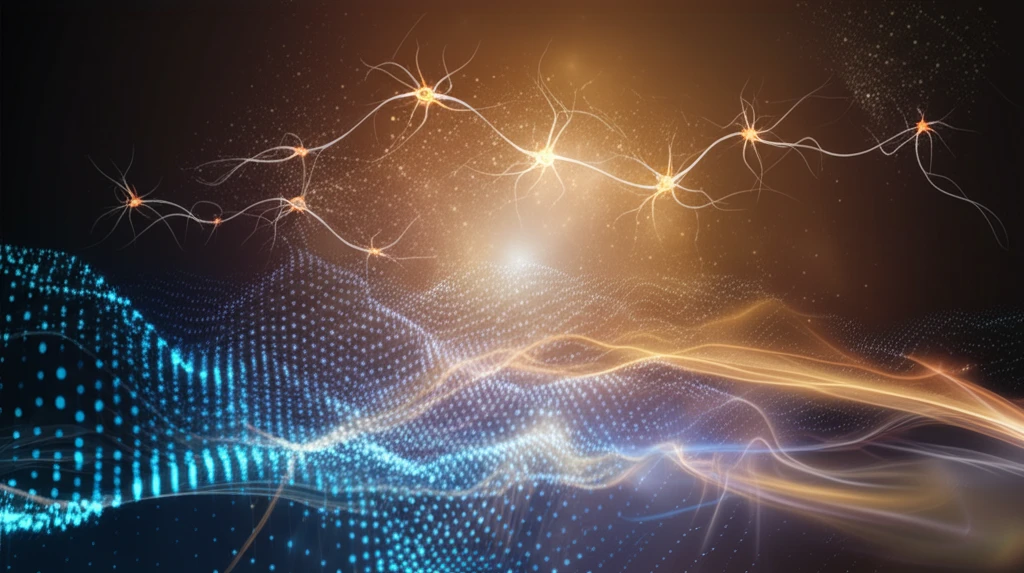
At its core, sentiment analysis aims to mimic the human ability to understand emotion from text. This is no easy task for a machine. Humans rely on a complex interplay of context, tone, and even shared cultural understanding to interpret feelings. AI systems need to be trained to recognize these subtle cues.
- Vocabulary Variations: Different fields or areas use different vocabularies and ways to convey specific sentiments. It's important for AI to adjust to each kind.
- Contextual Understanding:: AI needs to grasp the context of what's written to find what the true emotion is behind someone's words, just like we do when we talk to each other.
- Data Scarcity: The user-generated data has a variety of fields, each with their own training label. This has made it difficult to properly train.
The Future of Sentiment Analysis: Deeper Understanding, Broader Impact
AI-powered sentiment analysis is rapidly evolving, and its potential applications are vast. It promises to transform how businesses understand their customers, how political campaigns connect with voters, and how we all navigate the complex world of online information. As AI becomes even more sophisticated, we can expect even more nuanced and insightful understandings of human emotion in the digital sphere.